AI can’t steal your job if you work alongside it — here’s how we might collaborate
Whether it’s athletes on a sporting field or celebrities in the jungle, nothing holds our attention like the drama of vying for a single prize. And when it comes to the evolution of artificial intelligence (AI), some of the most captivating moments have also been delivered in nailbiting finishes.
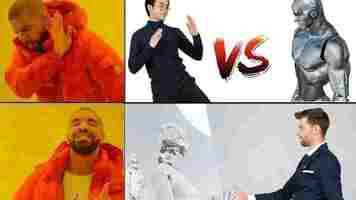
In 1997, IBM’s Deep Blue chess computer was pitted against grandmaster and reigning world champion Garry Kasparov, having lost to him the previous year.
But this time, the AI won. The popular Chinese game Go was next, in 2016, and again there was a collective intake of breath when Google’s AI was victorious . These competitions elegantly illustrate what is unique about AI: we can program it to do things we can’t do ourselves, such as beat a world champion.
But what if this framing obscures something vital – that human and artificial intelligence are not the same? AI can quickly process vast amounts of data and be trained to execute specific tasks; human intelligence is significantly more creative and adaptive.
The most interesting question is not who will win, but what can people and AI achieve together? Combining both forms of intelligence can provide a better outcome than either can achieve alone.
This is called collaborative intelligence. And this is the premise of CSIRO’s new A$12 million Collaborative Intelligence (CINTEL) Future Science Platform, which we are leading.
Checkmate mates
While chess has been used to illustrate AI-human competition, it also provides an example of collaborative intelligence. IBM’s Deep Blue beat the world champion, but did not render humans obsolete. Human chess players collaborating with AI have proven superior to both the best AI systems and human players.
And while such “freestyle” chess requires both excellent human skill and AI technology, the best results don’t come from simply combining the best AI with the best grandmaster. The process through which they collaborate is crucial.
So for many problems – particularly those that involve complex, variable and hard-to-define contexts – we’re likely to get better results if we design AI systems explicitly to work with human partners, and give humans the skills to interpret AI systems.
A simple example of how machines and people are already working together is found in the safety features of modern cars. Lane keep assist technology uses cameras to monitor lane markings and will adjust the steering if the car appears to be drifting out of its lane.
However, if it senses the driver is actively steering away, it will desist so the human remains in charge (and the AI continues to assist in the new lane). This combines the strengths of a computer, such as limitless concentration, with those of the human, such as knowing how to respond to unpredictable events.
There is potential to apply similar approaches to a range of other challenging problems. In cybersecurity settings, humans and computers could work together to identify which of the many threats from cybercriminals are the most urgent.
Similarly, in biodiversity science, collaborative intelligence can be used to make sense of massive numbers of specimens housed in biological collections.
Laying the foundations
We know enough about collaborative intelligence to say it has massive potential, but it’s a new field of research – and there are more questions than answers.
Through CSIRO’s CINTEL program we will explore how people and machines work and learn together, and how this way of collaborating can improve human work. Specifically, we will address four foundations of collaborative intelligence:
Robots reimagined
One of our projects will involve working with the CSIRO-based robotics and autonomous systems team to develop richer human-robot collaboration. Collaborative intelligence will enable humans and robots to respond to changes in real time and make decisions together.
For example, robots are often used to explore environments that might be dangerous for humans, such as in rescue missions. In June, robots were sent to help in search and rescue operations, after a 12-storey condo building collapsed in Surfside , Florida.
Often, these missions are ill-defined, and humans must use their own knowledge and skills (such as reasoning, intuition, adaptation and experience) to identify what the robots should be doing. While developing a true human-robot team may initially be difficult, it’s likely to be more effective in the long term for complex missions.
This article by Cecile Paris , Chief Research Scientist, Knowledge Discovery & Management, CSIRO and Andrew Reeson , Economist, Data61, CSIRO , is republished from The Conversation under a Creative Commons license. Read the original article .
Black teen misidentified by facial recognition sparks fears of machine-driven segregation
A 14-year-old Black girl has become another victim of a facial recognition failure.
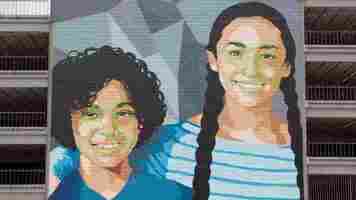
Lamya Robinson was kicked out of a skating rink in Michigan after a facial recognition system misidentified the teen as someone who’d been banned by the business.
The incident has escalated concerns about machine-driven segregation. But let’s dive into what exactly happened.
Not even skating is safe from facial recognition
When Robinson tried to enter the roller skating rink, staff stopped her because, they said, she had previously been involved in a fight at the venue. But the teenager had never even been there before.
The facial recognition system had incorrectly matched her face to another person.
“To me, it’s basically racial profiling,” her mother, Juliea Robinson, told Fox 2 Detroit . “You’re just saying every young Black, brown girl with glasses fits the profile and that’s not right.”
In a statement given to the TV channel, the rink said one of its managers had asked Juliea to call back sometime during the week:
The girl’s parents said they’re considering legal action against the rink.
Sadly, we shouldn’t be surprised
Facial recognition is notoriously prone to errors and biases. Numerous studies have demonstrated that the software discriminates on race and gender, with Black women particularly vulnerable to the biases.
The errors have already led to wrongful arrests . But experts warn that the software is also propagating segregation.
“When we say this is a civil rights issue it goes beyond false arrests, it’s about who gets to access public spaces in a world of machine-driven segregation,” tweeted Ángel Díaz, a counsel in the Liberty and National Security Program at the Brennan Center.
If algorithms are determining who can go where, they’ll inevitably restrict the rights of people that the software’s biased against. It’s another good reason to ban the use of facial recognition in public spaces.
Greetings Humanoids! Did you know we have a newsletter all about AI? You can subscribe to it right here .
We’ll never find dark matter… without quantum tech
Almost a century ago, Dutch astronomer Jacobus Kapteyn first proposed the existence of dark matter. He’d been studying the motion of stars in galaxies — a galaxy can be described, in rough terms, as a heap of stars, gas and dust rotating around a common center — and noticed that something was off. The stars in the outer layers of the galaxy were rotating much too fast to conform with the laws of gravity. Kapteyn’s hypothesis was that some invisible, massive stuff might be in and around the galaxy, making the outer stars reach the observed velocities.
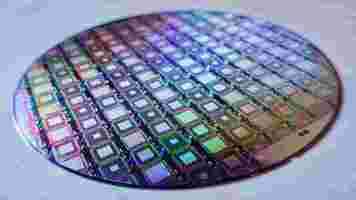
Fro m the 1960s to the ’80s, Vera Rubin, Kent Ford and Ken Freeman gathered more evidence in support of this hypothesis. They ultimately showed that most galaxies must contain six times as much invisible mass as they do visible stars, gas and dust.
Other observations in favor of dark matter followed, such as gravitational lensing and anisotropies in the cosmic microwave background. Gravitational lensing is a phenomenon in which light beams get bent around massive objects; the cosmic microwave background is an outer layer of the universe that would be quite homogeneous without dark matter but is rather lumpy in reality.
In the meantime, uncountable masterminds have devised theories and designed experiments to track down dark matter . Nevertheless, nobody has actually seen a dark matter particle as of now. That’s why, even today, senior scientists, as well as doctoral students like myself, are still conducting research on dark matter. And it still seems as though discovering dark matter is many centuries — maybe even millennia — away.
With recent advances in quantum computing, however, dark matter physics might experience a massive boost. The search for two types of dark matter — scientists don’t actually know whether they both exist but they’re trying to figure that out — might profit from quantum technology. The first type is the axion , whose existence might explain why the strong nuclear force doesn’t change if you flip a particle’s electric charge and parity. The other type is the dark photon. These particles would behave similarly to photons, the particles of light, except that dark photons aren’t light at all, of course.
Searching for axions
According to theory, axions should be wobbling through space and time at a particular frequency. The only problem is that theorists are unable to predict what that frequency might be. So, researchers are left to scan an enormous range of frequencies, one small band at a time.
Like an old radio receiver converts radio waves into sound, axion detectors convert axion waves into electromagnetic signals. This process gets more complicated, though, because axions oscillate at two different frequencies simultaneously.
You can picture this looking a little like a drunk person trying to get home from a party: They might take three steps to the right, then three steps to the left, then back to the right again. That’s one frequency, on the “left-right” spectrum. Because they also have massive hiccups, though, they might jump into the air at each HIC!, which occurs every four steps. That’s the second frequency, on the “up-down” spectrum.
Axions may be a little more sophisticated than drunk people, but they also have two frequencies, just like partygoers who have enjoyed a glass too many.
Mathematically, one can put these two frequencies together by quadratically adding them. That is, one multiplies the first frequency by itself, adds the second frequency multiplied by itself and then takes the square root.
In our drunkard’s example, three steps times themselves equal nine steps squared for the first frequency, four steps times themselves equal 16 steps squared for the second frequency, and together we get that the square root of nine steps squared plus 16 steps squared is five steps. This — in our example, five steps — is called electromagnetic field quadrature .
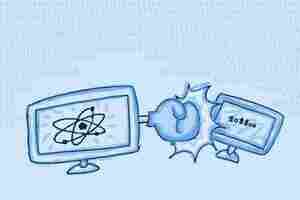
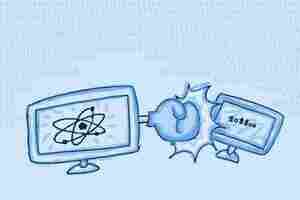
Now, usually, one would need to check a tiny band of frequencies (equal to one step in our example) over a huge range of frequencies (let’s say one to 200 steps). In reality, this is even harder. The axion frequency might be anywhere between 300 Hertz and 300 billion Hertz. That’s a big, fat range to cover! At the rate at which our current methods operate, covering this range may take up to 10,000 years because we can only test one small bandwidth at a time.
This bandwidth is limited by the so-called uncertainty principle . So, returning to our party going example, the observer might be drunk himself and therefore mis-count each frequency by two steps. They might miss two steps and calculate the square root of one squared plus two squared, which is roughly two steps. Or they might calculate the square root of five squared plus seven squared, which is roughly eight steps. That’s a bandwidth of six steps. So, if the observer wants to scan the range of one to 200 steps, they would have to measure 33 times. The larger the bandwidth, though, the less often they will need to measure the frequency.
This bandwidth can be enlarged, thankfully, through a process called quantum squeezing . Borrowing superconducting circuits straight from quantum computers, one can redistribute the uncertainties such that one frequency is more affected than the other.
In our example, the drunk observer might be able to count the up-down frequency quite well, to an accuracy of one step, but he might miss the left-right frequency by three steps. He might therefore measure anything between the square root of zero squared plus one squared, which is one step, to the square root of six squared plus five squared, which is roughly eight steps. The bandwidth has therefore increased by one step, and the observer only needs to measure 28 instead of 33 times.
The HAYSTAC collaboration, consisting of researchers at Yale University, the University of California–Berkeley, Lawrence Berkeley National Laboratory and the University of Colorado–Boulder, has implemented exactly this protocol. Though quantum squeezing, they’ve already cut the time of observation, previously 10,000 years, in half. With further improvements, they believe they can get the search for axions up to 10 times faster.
Letting dark photons travel through walls
At Fermilab, scientists are working on speeding up the search for dark photons. For this search, they fill one superconducting microwave cavity with photons and keep another as empty as possible. According to theory, a small fraction of these photons should spontaneously turn into dark photons. Since dark photons can travel through walls (yes, wild stuff), some of these will end up in a second cavity that the scientists set up. A small fraction of these dark photons would then turn back into regular photons, which scientists can then detect .
There are two problems with these photon-detectors, though. First, they return false positives every so often. That is, they indicate that they’ve found a photon although, in reality, no photon is in the trap! Second, when a photon enters the detector, it’s gone. That means that it’s impossible to check whether a positive result is false positive or a true one.
Quantum measurements circumvent both problems. They conserve the photons, meaning that they can repeat a measurement until the end of a photon’s natural lifetime. And by measuring repeatedly, false positive results can be eliminated. If no photon is there and the detector shows positive nevertheless, the chances are quite high that it will show negative upon subsequent measurements. That’s because the chances of the detector showing positive when a photon is around is higher than when there’s none around (as it should be), so showing a string of false positives is quite unlikely.
In addition, Fermilab’s expertise in building cavities pays off by prolonging the lifetimes of photons. Essentially, in low-quality cavities, photons disappear quickly. In Fermilab’s cavities, however, they survive a long time. This durability makes it easier to repeat measurements again and again.
By combining expertise in cavities and quantum measurements, this technique is 36 times more sensitive than the quantum limit, i the benchmark of conventional quantum measurements. Without quantum techniques, detecting dark photons might even be impossible.
The search is heating up
Physicists have already spent almost a century on dark matter, and there are no signs that this mystery will be solved soon. Nevertheless, the search is making progress.
What’s especially exciting is that quantum tech, be it quantum squeezing, quantum measurements or maybe even quantum computing, is being applied in these searches. Detection methods that are still in their infancy are being deployed in the quest to find the most elusive particles to date. To find invisible particles, scientists are using and developing the technology of the future.
Dark matter has already got a long and rich history. You could spend half a lifetime just studying this, from its first conceptions to decades-worth of experiments that have failed to find it. But scientists aren’t reluctant to add another chapter or two or even 10 that might be even more exciting than the previous ones. Perhaps cutting-edge quantum tech will be what finally breaks the mystery.
This article was written by Ari Joury and was originally published on Towards Data Science . You can read it here .