Here’s how OpenAI’s magical DALL-E image generator works
It seems like every few months, someone publishes a machine learning paper or demo that makes my jaw drop. This month, it’s OpenAI’s new image-generating model, DALL·E .
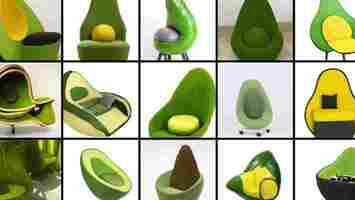
This behemoth 12-billion-parameter neural network takes a text caption (i “an armchair in the shape of an avocado”) and generates images to match it:
I think its pictures are pretty inspiring (I’d buy one of those avocado chairs), but what’s even more impressive is DALL·E’s ability to understand and render concepts of space, time, and even logic (more on that in a second).
In this post, I’ll give you a quick overview of what DALL·E can do, how it works, how it fits in with recent trends in ML, and why it’s significant. Away we go!
What is DALL·E and what can it do?
In July, DALL·E’s creator, the company OpenAI, released a similarly huge model called GPT-3 that wowed the world with its ability to generate human-like text , including Op Eds, poems, sonnets, and even computer code. DALL·E is a natural extension of GPT-3 that parses text prompts and then responds not with words but in pictures. In one example from OpenAI’s blog, for example, the model renders images from the prompt “a living room with two white armchairs and a painting of the colosseum. The painting is mounted above a modern fireplace”:
Pretty slick, right? You can probably already see how this might be useful for designers. Notice that DALL·E can generate a large set of images from a prompt. The pictures are then ranked by a second OpenAI model, called CLIP , that tries to determine which pictures match best.
How was DALL·E built?
Unfortunately, we don’t have a ton of details on this yet because OpenAI has yet to publish a full paper. But at its core, DALL·E uses the same new neural network architecture that’s responsible for tons of recent advances in ML: the Transformer . Transformers, discovered in 2017, are an easy-to-parallelize type of neural network that can be scaled up and trained on huge datasets. They’ve been particularly revolutionary in natural language processing (they’re the basis of models like BERT, T5, GPT-3, and others), improving the quality of Google Search results, translation, and even in predicting the structures of proteins .
Most of these big language models are trained on enormous text datasets (like all of Wikipedia or crawls of the web ). What makes DALL·E unique, though, is that it was trained on sequences that were a combination of words and pixels. We don’t yet know what the dataset was (it probably contained images and captions), but I can guarantee you it was probably massive.
How “smart” is DALL·E?
While these results are impressive, whenever we train a model on a huge dataset, the skeptical machine learning engineer is right to ask whether the results are merely high-quality because they’ve been copied or memorized from the source material.
To prove DALL·E isn’t just regurgitating images, the OpenAI authors forced it to render some pretty unusual prompts:
“A professional high quality illustration of a giraffe turtle chimera.”
“A snail made of a harp.”
It’s hard to imagine the model came across many giraffe-turtle hybrids in its training data set, making the results more impressive.
What’s more, these weird prompts hint at something even more fascinating about DALL·E: its ability to perform “zero-shot visual reasoning.”
Zero-Shot Visual Reasoning
Typically, in machine learning, we train models by giving them thousands or millions of examples of tasks we want them to preform and hope they pick up on the pattern.
To train a model that identifies dog breeds, for example, we might show a neural network thousands of pictures of dogs labeled by breed and then test its ability to tag new pictures of dogs. It’s a task with limited scope that seems almost quaint compared to OpenAI’s latest feats.
Zero-shot learning, on the other hand, is the ability of models to perform tasks that they weren’t specifically trained to do. For example, DALL·E was trained to generate images from captions. But with the right text prompt, it can also transform images into sketches:
DALL·E can also render custom text on street signs:
In this way, DALL·E can act almost like a Photoshop filter, even though it wasn’t specifically designed to behave this way.
The model even shows an “understanding” of visual concepts (i “macroscopic” or “cross-section” pictures), places (i “a photo of the food of china”), and time (“a photo of alamo square, san francisco, from a street at night”; “a photo of a phone from the 20s”). For example, here’s what it spit out in response to the prompt “a photo of the food of china”:
In other words, DALL·E can do more than just paint a pretty picture for a caption; it can also, in a sense, answer questions visually.
To test DALL·E’s visual reasoning ability, the authors had it take a visual IQ test. In the examples below, the model had to complete the lower right corner of the grid, following the test’s hidden pattern.
“DALL·E is often able to solve matrices that involve continuing simple patterns or basic geometric reasoning,” write the authors, but it did better at some problems than others. When the puzzles’s colors were inverted, DALL·E did worse–“suggesting its capabilities may be brittle in unexpected ways.”
What does it mean?
What strikes me the most about DALL·E is its ability to perform surprisingly well on so many different tasks, ones the authors didn’t even anticipate:
“We find that DALL·E […] is able to perform several kinds of image-to-image translation tasks when prompted in the right way.
We did not anticipate that this capability would emerge, and made no modifications to the neural network or training procedure to encourage it.”
It’s amazing, but not wholly unexpected; DALL·E and GPT-3 are two examples of a greater theme in deep learning: that extraordinarily big neural networks trained on unlabeled internet data (an example of “self-supervised learning”) can be highly versatile, able to do lots of things weren’t specifically designed for.
Of course, don’t mistake this for general intelligence. It’s not hard to trick these types of models into looking pretty dumb. We’ll know more when they’re openly accessible and we can start playing around with them. But that doesn’t mean I can’t be excited in the meantime.
This article was written by Dale Markowitz , an Applied AI Engineer at Google based in Austin, Texas, where she works on applying machine learning to new fields and industries. She also likes solving her own life problems with AI, and talks about it on YouTube.
Here’s what all successful AI startups have in common
With tech giants pouring billions of dollars into artificial intelligence projects, it’s hard to see how startups can find their place and create successful business models that leverage AI. However, while fiercely competitive, the AI space is also constantly causing fundamental shifts in many sectors. And this creates the perfect environment for fast-thinking and -moving startups to carve a niche for themselves before the big players move in.
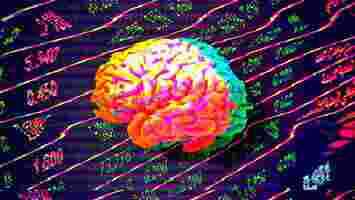
Last week, technology analysis firm CB Insights published an update on the status of its list of top 100 AI startups of 2020 (in case you don’t know, CB Insight publishes a list of 100 most promising AI startups every year). Out of the hundred startups, four have made exits, with three going public and one being acquired by Facebook.
A closer look at these startups provides some good hints at what it takes to create a successful business that makes use of AI. And (un)surprisingly, artificial intelligence is a small part — albeit an important one — of a successful product management strategy. Here are some of the key takeaways from AI startups that have managed to reach a stable status.
Lemonade: AI complements a successful product strategy
Lemonade, an insurtech startup founded in 2015, made its initial public offering in July with a $1.7 billion valuation. Lemonade is an online platform that aims to address some of the key problems of the traditional home insurance industry. The company has been able to develop its business through smart design and a good marketing strategy. The AI component was built on top of that.
The company’s website and mobile app are very easy to use. The process of buying insurance and filing claims with the app and website goes through digital assistants and is much faster than traditional insurance companies. As one of the first movers in the insurtech space, Lemonade had the edge over other similar companies that have cropped up in recent years, and it was able to quickly snatch a lot of users who were looking for a shift from traditional insurance model to one that was more tech-focused.
Lemonade’s business model and messaging are also interesting. The company takes a flat fee from premiums, which means the company doesn’t make a profit from denying claims. The unclaimed money goes to charities of users’ choice. The company also says that it will not invest premiums into heavily polluting industries and companies that cause harm. So, basically, Lemonade is marketing itself as the good guy in a historically reviled industry, on a mission, per the company’s words, to “transform insurance from a necessary evil to a social good.”
Insurance depends a lot on data, and established agencies have more than a century of data they can use to develop risk models and create insurance policies. Lemonade didn’t have the data of traditional agencies, but it also didn’t have their baggage of customers and old policies. It was able to create its entire technology stack from the ground up to cater to the needs of an AI factory .
With the entire experience being digitized, the company can collect a lot more data from each customer interaction, including data points that other agencies do not capture. This enables the company to create machine learning models that not only predict insurance risk with growing accuracy over time but can also create automation and personalization opportunities that were impossible before. The company has two AI chatbots: Maya helps you create your insurance plan in a few minutes, and Jim handles the claims process. According to the company, AI handles a third of the cases and pays claims in a matter of minutes. The rest of the claims are transferred to human agents. The chatbot continues to improve as it gathers more data.
The company believes that with time, the AI will give it the edge over traditional agencies and allow it to provide much more affordable plans to customers. And its $480 million pre-IPO funding and its post-IPO growth show that investors believe its plan can work.
Lemonade’s head start is its biggest protection. Other startups that would want to copy its business model don’t have its data and can’t create equally efficient AI models. And it has also created a protective moat against traditional insurance agencies, which are much slower to move into new areas. By the time they do create their own AI factories, Lemonade will have carved a comfortable niche for itself.
Butterfly Network: Specialized hardware with AI enhancements
Butterfly Network will be listed on the New York Stock exchange after a $1.5 billion special purpose acquisition company (SPAC) merger with Longview Capital later this year.
The company’s product is Butterfly iQ, a medically approved single-probe, whole-body ultrasound device that connects to a smartphone and works with an accompanying mobile app. The device costs $2,000, which is much more affordable than the five- and six-digit-priced ultrasound sets usually found at hospitals. The company aims to make high-quality ultrasound imaging available to communities that can’t afford high-end devices and bring portable scanning to places where the bulky ultrasound sets can’t go.
iQ also uses artificial intelligence to create use cases that are not available on other ultrasound devices. For instance, one of the AI features of iQ is a slider in the app that shows the quality of the image to the user. As the user moves the probe on the patient’s body, the slider shifts to show whether the device is getting a good capture or not. The feature uses an artificial neural network that has been trained on tens of thousands of images to discriminate between good and bad images. For instance, frontline responders or clinics whose staff don’t have the expertise with ultrasound can use the device to get proper images and send them to experts for further analysis.
The device and app come bundled with a bunch of cloud storage and sharing features that facilitate the use of data in a broader health care context.
The company is also working to add new machine learning-powered features to help with measurement and analysis.
So here too, I think that AI is a small but important part of the overall business. The biggest value comes from the hardware. The small, portable ultrasound device allows Butterfly to differentiate itself from other manufacturers and create value for untapped segments of the market. AI is the added value that helps it improve the software stack that builds on top of the hardware. Given that the device uses consumer smartphones, it also has the potential to add new AI features and continually improve its product’s performance as mobile device hardware becomes better.
The one risk I see in Butterfly’s AI business is the possibility of similar moves from household names such as Philips and Siemens. Should health tech giants decide to enter the handheld ultrasound business, Butterfly Network will need to find something that can protect its products against copycats. One possible solution would be for Butterfly to work out a privacy-friendly plan to collect ultrasound data from iQ devices to improve the performance of its AI models. But it will not be very easy, given the sensitive nature of health data.
C3.ai: Enterprise AI can work if you have the reputation
C3.ai, another one of the successful AI startups mentioned by CB Insights, is a provider of enterprise AI software. C3.ai’s pre-IPO valuation was $4 billion, but on the first day of trading, its market cap skyrocketed above $13 billion.
C3.ai software helps companies build AI models on top of their data for predictive maintenance, improved inventory management, fraud detection, energy management, and other operational enhancements that can reduce costs and increase productivity. C3.ai is not a provider of cloud services but its software is compatible with most top cloud providers such as Microsoft Azure, Amazon Web Services, Google Cloud, and IBM Cloud.
Under normal circumstances, C3.ai’s product strategy would be considered risky. From a technical standpoint, it has no key differentiator. It is providing services that can easily be replicated by another company that has the right resources, including the very cloud services its software integrates with. And since its founding in 2009, the company has changed its name twice from C3 Energy to C3 IoT and then to C3.ai, which sounds a bit opportunistic.
What makes C3.ai different, however, is its founder Thomas Siebel, a billionaire and a well-known and respected entrepreneur. C3.ai’s success hinges not on a lot of small customers but on creating ripple effects in different sectors by acquiring big customers. In this respect, having a person on board who has the reputation and experience of Siebel can make a big difference. Currently, C3.ai’s customers include machinery manufacturer Caterpillar, oil and gas services company Baker Hughes, and energy company Engie, all big names in their respective industries. Interestingly, 36 percent of its revenue in 2020 came from Baker Hughes and Engie.
Therefore, although C3.ai provides very good AI development tools, the company’s success can be largely attributed not to its unique AI capabilities but its customer acquisition and retention strategy. I’m not sure if that would have been possible without having someone at the helm of the company who has strong connections in different markets and a reputation for delivering great products.
Mapillary: The value of data
The final company that’s worth examining in the CB Insights list is Mapillary, acquired by Facebook in June for an undisclosed amount. Mapillary launched in 2013 to create a massive dataset of street-level images, rivaling Google’s Street View service.
Since its founding, Mapillary has collected more than one billion high-resolution images from cities around the world. Before being acquired by Facebook, Mapillary had partnered with Amazon’s AI platform to extract information from images through computer vision .
Mapillary didn’t have a super-advanced AI application or a very promising roadmap to making a profit over its data. But its data and services could prove to be a great addition to a larger ecosystem of AI software, such as that of Facebook. There are many ways Facebook, which is in the business of knowing more and more about its users, can turn a profit from Mapillary’s data. For now, we know that it will be integrating Mapillary’s data and applications into Facebook’s augmented reality and Marketplace platforms. And there are many other uses Facebook’s AI research unit can have for exclusive access to this large data set of labeled street images.
Therefore, I don’t quite see Mapillary as an AI success story, but its acquisition highlights the value of data in the AI industry. Large tech companies are often in search of ways to obtain exclusive data to hone their AI models and gain an edge over competitors. And they’re more than willing to take a shortcut by purchasing another company’s data—and perhaps the whole company with it.
The “AI startup” misnomer
I think “AI startup” is a misnomer when applied to many of the companies included in the CB Insights list because it puts too much focus on the AI side and too little on the other crucial aspects of the company.
Successful companies start by addressing an overlooked or poorly solved problem with a sound product strategy. This gives them the minimum market penetration needed to establish their business model and gather data to gain insights, steer their product in the right direction, and train machine learning models. Finally, they use AI as a differentiating factor to solidify their position and maintain the edge over competitors.
No matter how advanced, AI algorithms alone don’t make a successful startup nor a business strategy.
This article was originally published by Ben Dickson on TechTalks , a publication that examines trends in technology, how they affect the way we live and do business, and the problems they solve. But we also discuss the evil side of technology, the darker implications of new tech and what we need to look out for. You can read the original article here .
Scientists think there could be alien life on one of Jupiter’s moons
There’s two things you should know about Jupiter. First, it would be one helluva planet to live on if you were a werewolf. That’s because it has 79 moons. Second, one of those moons probably has life on it.
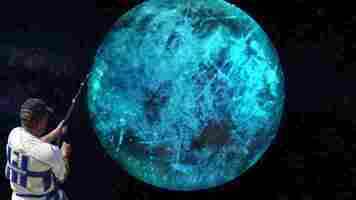
We say probably because, based on all the evidence, it would be weird if it didn’t.
Scientists have long thought Europa, a small icy moon about a quarter the size of Earth, might contain life. After all, it’s supposedly got everything you need to sustain biology as we know it: oxygen, water, and nutrients.
But there’s always been one hitch: Europa’s oxygen and its water are separated by a thick sheet of ice. And, until now, nobody’s been able to hypothesize a way for that oxygen to supply any potential life in the watery parts of the moon’s oceans.
A team of scientists led by Mark Hesse of the University of Austin recently conducted simulations demonstrating a theoretical method by which oxygen could actually penetrate Europa’s ice shell and reach the water beneath.
According to the team’s research paper :
In essence, the team asserts that salty brine could occasionally form rivulets of draining oxygen from the ice shelf that might allow a significant portion of Europa’s surface oxygen to escape into the under-ocean.
Whew, that seems like a lot of conjecture. But there’s good reason to be excited: if the scientists are right, then Europa is extremely well-suited as a candidate for alien life.
Despite the fact it’s a quarter the size of our planet, its oceans are suspected to be at least twice as big and many times deeper. In fact, many scientists believe they might extend all the way to the planet’s core.
In scientific terms, this is a jackpot. There should be plenty of cast-off molecules captured beneath the planet’s surface to serve as potential nutrients and the myriad chemical reactions that could occur at the seam between the moon’s core and its oceans could make for a perfect simmering pan for the primordial soup of life.
Toss in the fact that these oceans have a protective shell that, according to the new research, could keep harmful radiation out while still allowing oxygen to filter through, and it feels like a near-certainty we should find some signs of life on the planet.
Unfortunately, what we see in simulations is a lot harder to reproduce in the real world. In order to truly determine once-and-for-all whether Europa hosts life, we’ll need to drill at least a foot beneath the surface before we could hope to detect even the most ancient signs.
And, if life still exists on the moon, we’d likely have to go significantly deeper to observe a living specimen.
Currently, NASA has plans to send a vessel to Europa in 2024 to scout out spots for a potential drilling mission in the future.
In the meantime, the organization is busy scrambling to meet its self-inflicted deadlines for crewed missions to Earth’s moon and Mars.