How an AI brain with only one neuron could surpass humans
A multi-disciplinary team of researchers from Technische Universität Berlin recently created a neural ‘network’ that could one day surpass human brain power with a single neuron.
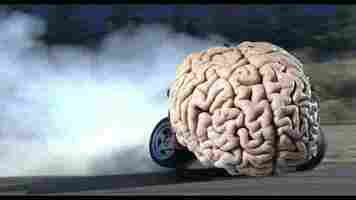
Our brains have approximately 86 billion neurons. Combined, they make up one of the most advanced organic neural networks known to exist.
Current state-of-the-art artificial intelligence systems attempt to emulate the human brain through the creation of multi-layered neural networks designed to cram as many neurons in as little space as possible.
Unfortunately, such designs require massive amounts of power and produce outputs that pale in comparison to the robust, energy-efficient human brain.
Per an article from The Register’s Katyanna Quach, scientists estimate the costs for training just one neural “super network” to exceed that of a nearby space mission:
The Berlin team decided to challenge the idea that bigger is better by building a neural network that uses a single neuron.
Typically, a network needs more than one node. In this case however, the single neuron is able to network with itself by spreading out over time instead of space.
Per the team’s research paper :
In a traditional neural network, such as GPT-3, each neuron can be weighted in order to fine-tune results. The result, typically, is that more neurons produce more parameters, and more parameters produce finer results.
But the Berlin team figured out that they could perform a similar function by weighting the same neuron differently over time instead of spreading differently-weighted neurons over space.
Per a press release from Technische Universität Berlin:
“Rapidly” is putting it mildly though. The team says their system can theoretically reach speeds approaching the universe’s limit by instigating time-based feedback loops in the neuron via lasers — neural networking at or near the speed of light.
What does this mean for AI? According to the researchers, this could counter the rising energy costs of training strong networks. Eventually we’re going to run out of feasible energy to use if we continue to double or triple usage requirements with bigger networks over time.
But the real question is whether a single neuron stuck in a time loop can produce the same results as billions.
In initial testing, the researchers used the new system to perform computer vision functions. It was able to remove manually-added noise from pictures of clothing in order to produce an accurate image — something that’s considered fairly advanced for modern AI.
With further development, the scientists believe the system could be expanded to create “a limitless number” of neuronal connections from neurons suspended in time.
It’s feasible such a system could surpass the human brain and become the world’s most powerful neural network, something AI experts refer to as a “ superintelligence .”
Military experts fear the Pentagon’s been sucked into the AI hype machine
A pair of military experts recently penned an article with a simple warning to the Pentagon: winter is coming.
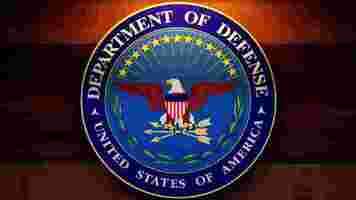
In this case, said winter is an artificial intelligence winter, and said AI winter is an artificial AI winter.
To put it another way: the Pentagon’s shooting itself in the foot while everyone else is aiming down-range.
The gist: The authors of the article are Marc Losito and John Anderson, a pair of military experts (the former’s a career Special Forces soldier currently on active duty and the latter’s a reserve officer who specializes in applying AI and machine learning to military applications). They believe that the Pentagon’s guilty of believing too much hype when it comes to AI.
Per the article :
Background: The big idea here is that the Pentagon’s always invested in military technology research and development. These experts aren’t asking the government to stop, they’re warning the government against treating AI like any other technology.
The writers warn that the US has been here before when it comes to AI. After WWII, for example, US military leaders were pretty high on the idea of replacing soldiers with machines that could fight for themselves. In retrospect it’s easy to demonstrate that computer technology wasn’t nearly advanced enough to pull off robust autonomous combat units beyond experimental toys.
The problem: The calculus has changed. The Pentagon is no longer viewing AI in narrow, project-specific terms. It’s going all-in on AI as a method to revolutionize the way we fight wars.
As history has taught us, the most revolutionary war tools are seldom developed during times of peaceful discovery. They’re usually forged in the fires of necessity.
The warning : The experts don’t appear to be concerned with the surface risks of budget misappropriation or the government simply being duped by bad actors selling them overhyped AI systems. They’re more worried about the existential threat of the US military losing its taste for AI once all the junk it’s paid for doesn’t pan out.
These experts appear to be warning us that the US isn’t properly testing and vetting these programs against the real-world needs of boots-on-the-ground assets in the ever-evolving battlespace.
Per the article:
Quick take: What’s the worst that could happen? Whiplash. The experts fear the response to the Pentagon’s failure to deliver on its hyperbolic promises for the future of AI in warfare will cause military leadership to lose faith in its own programs.
An over-correction like that could indeed cause an AI winter for the US while everyone else enjoys what the authors refer to as an AI spring.
What 100 suicide notes taught us about creating more empathetic chatbots
While the art of conversation in machines is limited, there are improvements with every iteration. As machines are developed to navigate complex conversations, there will be technical and ethical challenges in how they detect and respond to sensitive human issues.
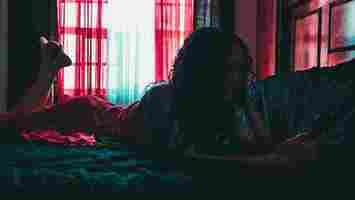
Our work involves building chatbots for a range of uses in health care. Our system, which incorporates multiple algorithms used inartificial intelligence (AI) and natural language processing, has been in development at the Australian e-Health Research Centre since 2014.
The system has generated several chatbot apps which are being trialed among selected individuals, usually with an underlying medical condition or who require reliable health-related information.
They include HARLIE for Parkinson’s disease and Autism Spectrum Disorder , Edna for people undergoing genetic counselling, Dolores for people living with chronic pain, and Quin for people who want to quit smoking.
Research has shown those people with certain underlying medical conditions are more likely to think about suicide than the general public. We have to make sure our chatbots take this into account.
We believe the safest approach to understanding the language patterns of people with suicidal thoughts is to study their messages. The choice and arrangement of their words, the sentiment and the rationale all offer insight into the author’s thoughts.
For our recent work we examined more than 100 suicide notes from various texts and identified four relevant language patterns: negative sentiment, constrictive thinking, idioms and logical fallacies.
Read more: Introducing Edna: the chatbot trained to help patients make a difficult medical decision
Negative sentiment and constrictive thinking
As one would expect, many phrases in the notes we analyzed expressed negative sentiment such as:
There was also language that pointed to constrictive thinking. For example:
The phenomenon of constrictive thoughts and language is well documented . Constrictive thinking considers the absolute when dealing with a prolonged source of distress.
For the author in question, there is no compromise. The language that manifests as a result often contains terms such as either/or, always, never, forever, nothing, totally, all and only .
Language idioms
Idioms such as “the grass is greener on the other side” were also common — although not directly linked to suicidal ideation. Idioms are often colloquial and culturally derived, with the real meaning being vastly different from the literal interpretation.
Such idioms are problematic for chatbots to understand. Unless a bot has been programmed with the intended meaning, it will operate under the assumption of a literal meaning.
Chatbots can make some disastrous mistakes if they’re not encoded with knowledge of the real meaning behind certain idioms. In the example below, a more suitable response from Siri would have been to redirect the user to a crisis hotline.
The fallacies in reasoning
Words such as therefore, ought and their various synonyms require special attention from chatbots. That’s because these are often bridge words between a thought and action. Behind them is some logic consisting of a premise that reaches a conclusion, such as :
This closely resemblances a common fallacy (an example of faulty reasoning) called affirming the consequent . Below is a more pathological example of this, which has been called catastrophic logic :
This is an example of a semantic fallacy (and constrictive thinking) concerning the meaning of I , which changes between the two clauses that make up the second sentence.
This fallacy occurs when the author expresses they will experience feelings such as happiness or success after completing suicide — which is what this refers to in the note above. This kind of “autopilot” mode was often described by people who gave psychological recounts in interviews after attempting suicide.
Preparing future chatbots
The good news is detecting negative sentiment and constrictive language can be achieved with off-the-shelf algorithms and publicly available data. Chatbot developers can (and should) implement these algorithms.
Generally speaking, the bot’s performance and detection accuracy will depend on the quality and size of the training data. As such, there should never be just one algorithm involved in detecting language related to poor mental health.
Detecting logic reasoning styles is a new and promising area of research . Formal logic is well established in mathematics and computer science, but to establish a machine logic for commonsense reasoning that would detect these fallacies is no small feat.
Here’s an example of our system thinking about a brief conversation that included a semantic fallacy mentioned earlier. Notice it first hypothesizes what this could refer to, based on its interactions with the user.
Although this technology still requires further research and development, it provides machines a necessary — albeit primitive — understanding of how words can relate to complex real-world scenarios (which is basically what semantics is about).
And machines will need this capability if they are to ultimately address sensitive human affairs — first by detecting warning signs, and then delivering the appropriate response.
This article by David Ireland , Senior Research Scientist at the Australian E-Health Research Centre., CSIRO and Dana Kai Bradford , Principal Research Scientist, Australian eHealth Research Centre, CSIRO , is republished from The Conversation under a Creative Commons license. Read the original article .