How knowledge distillation compresses neural networks
If you’ve ever used a neural network to solve a complex problem, you know they can be enormous in size, containing millions of parameters. For instance, the famous BERT model has about ~110 million.
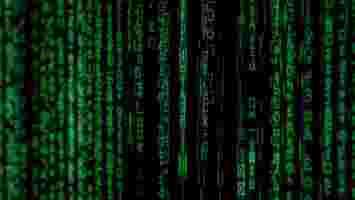
To illustrate the point, this is the number of parameters for the most common architectures in (natural language processing) NLP, as summarized in the recent State of AI Report 2020 by Nathan Benaich and Ian Hogarth . You can see this below:
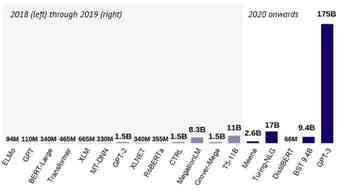
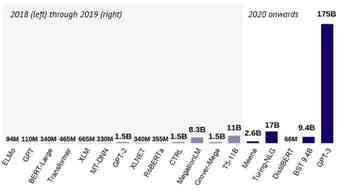
In Kaggle competitions, the winner models are often ensembles, composed of several predictors. Although they can beat simple models by a large margin in terms of accuracy, their enormous computational costs make them utterly unusable in practice.
Is there any way to somehow leverage these powerful but massive models to train state of the art models, without scaling the hardware?
Currently, there are three main methods out there to compress a neural network while preserving the predictive performance:
Weight pruning
Quantization
Knowledge distillation
In this post, my goal is to introduce you to the fundamentals of knowledge distillation , which is an incredibly exciting idea, building on training a smaller network to approximate the large one.
So, what is knowledge distillation?
Let’s imagine a very complex task, such as image classification for thousands of classes. Often, you can’t just slap on a ResNet50 and expect it to achieve 99% accuracy. So, you build an ensemble of models, balancing out the flaws of each one. Now you have a huge model, which, although performs excellently, there is no way to deploy it into production and get predictions in a reasonable time.
However, the model generalizes pretty well to the unseen data, so it is safe to trust its predictions. (I know, this might not be the case, but let’s just roll with the thought experiment for now.)
What if we use the predictions from the large and cumbersome model to train a smaller, so-called student model to approximate the big one?
This is knowledge distillation in essence, which was introduced in the paper Distilling the Knowledge in a Neural Network by Geoffrey Hinton, Oriol Vinyals, and Jeff Dean.
In broad strokes, the process is the following.
Train a large model that performs and generalizes very well. This is called the teacher model .
Take all the data you have, and compute the predictions of the teacher model. The total dataset with these predictions is called the knowledge, and the predictions themselves are often referred to as soft targets . This is the knowledge distillation step.
Use the previously obtained knowledge to train the smaller network, called the student model .
To visualize the process, you can think of the following.
Let’s focus on the details a bit. How is the knowledge obtained?
In classifier models, the class probabilities are given by a softmax layer, converting the logits to probabilities:
The logits produced by the last layer. Instead of these, a slightly modified version is used:
Where T is a hyperparameter called temperature . These values are called soft targets .
If T is large, the class probabilities are “softer”, that is, they will be closer to each other. In the extreme case, when T approaches infinity,
If T = 1 , we obtain the softmax function. For our purposes, the temperature is set to higher than 1, thus the name distillation .
Hinton, Vinyals, and Dean showed that a distilled model can perform as good as an ensemble composed of 10 large models.
Why not train a small network from the start?
You might ask, why not train a smaller network from the start? Wouldn’t it be easier? Sure, but it wouldn’t work necessarily.
Empirical evidence suggests that more parameters result in better generalization and faster convergence. For instance, this was studied by Sanjeev Arora, Nadav Cohen, and Elad Hazan in their paper On the Optimization of Deep Networks: Implicit Acceleration by Overparameterization .
For complex problems, simple models have trouble learning to generalize well on the given training data. However, we have much more than the training data: the teacher model’s predictions for all the available data.
This benefits us in two ways.
First, the teacher model’s knowledge can teach the student model how to generalize via available predictions outside the training dataset. Recall that we use the teacher model’s predictions for all available data to train the student model, instead of the original training dataset.
Second, the soft targets provide more useful information than class labels: it indicates if two classes are similar to each other . For instance, if the task is to classify dog breeds, information like “Shiba Inu and Akita are very similar” is extremely valuable regarding model generalization.
The difference between transfer learning
As noted by Hinton et al. , one of the earliest attempts to compress models by transferring knowledge was to reuse some layers of a trained ensemble, as done by Cristian Buciluǎ, Rich Caruana, and Alexandru Niculescu-Mizil in their 2006 paper titled Model compression .
In the words of Hinton et al.,
“…we tend to identify the knowledge in a trained model with the learned parameter values and this makes it hard to see how we can change the form of the model but keep the same knowledge. A more abstract view of the knowledge, that frees it from any particular instantiation, is that it is a learned mapping from input vectors to output vectors.” — Distilling the Knowledge in a Neural Network
Thus, the knowledge distillation doesn’t use the learned weights directly, as opposed to transfer learning.
Using decision trees
If you want to compress the model even further, you can try using even simpler models like decision trees. Although they are not as expressive as neural networks, their predictions can be explained by looking at the nodes individually.
This was done by Nicholas Frosst and Geoffrey Hinton, who studied this in their paper Distilling a Neural Network Into a Soft Decision Tree .
They showed that distilling indeed helped a little, although even simpler neural networks have outperformed them. On the MNIST dataset, the distilled decision tree model achieved 96.76% test accuracy, which was an improvement from the baseline 94.34% model. However, a straightforward two-layer deep convolutional network still reached 99.21% accuracy. Thus, there is a trade-off between performance and explainability.
Distilling BERT
So far, we have only seen theoretical results instead of practical examples. To change this, let’s consider one of the most popular and useful models in recent years: BERT.
Originally published in the paper BERT: Pre-training of Deep Bidirectional Transformers for Language Understanding by Jacob Devlin et al. from Google, it soon became widely used for various NLP tasks like document retrieval or sentiment analysis. It was a real breakthrough, pushing state of the art in several fields.
There is one issue, however. BERT contains ~110 million parameters and takes a lot of time to train. The authors reported that the training required 4 days using 16 TPU chips in 4 pods. Calculating with the currently available TPU pod pricing per hour , training costs would be around 10000 USD, not mentioning the environmental costs like carbon emissions .
One successful attempt to reduce the size and computational cost of BERT was made by Hugging Face . They used knowledge distillation to train DistilBERT , which is 60% the original model’s size while being 60% faster and keeping 97% of its language understanding capabilities.
The smaller architecture requires much less time and computational resources: 90 hours on 8 16GB V100 GPUs.
If you are interested in more details, you can read the original paper DistilBERT, a distilled version of BERT: smaller, faster, cheaper and lighter , or the summarizing article was written by one of the authors. This is a fantastic read, so I strongly recommend you to do so.
Conclusion
Knowledge distillation is one of the three main methods to compress neural networks and make them suitable for less powerful hardware.
Unlike weight pruning and quantization, the other two powerful compression methods, knowledge distillation does not reduce the network directly. Rather, it uses the original model to train a smaller one called the student model . Since the teacher model can provide its predictions even on unlabelled data, the student model can learn how to generalize like the teacher.
Here, we have looked at two key results: the original paper, which introduced the idea, and a follow-up, showing that simple models such as decision trees can be used as student models.
Google News probably thinks I cover Spiderman because algorithms are dumb
Google News holds a special place in the world of journalism. When multiple media outlets report on the same topic in a short amount of time, the articles that make it to the main News page are seen by the most people.
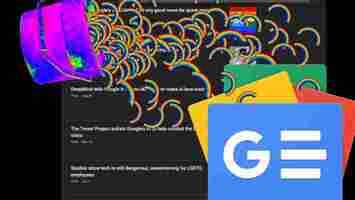
It’s no different than any other media industry. If you’re a musician, you want your song to show up on Spotify’s main page. If you’re in a comedy movie, you want it to be listed first in the “comedy” section on Netflix.
That’s why one of my crowning achievements as a journalist was convincing the Google News algorithm I was the queerest artificial intelligence reporter in the world.
How it started
Back in June I published this article explaining how my TNW author profile was influencing the number of my articles that showed up in Google News if you did an in-app search for “artificial intelligence queer.”
At the time, it was relevant because it was Pride month and a lot of people were covering how AI technologies impact the queer community.
When I casually searched for “artificial intelligence queer” to see what my peers were writing on the topic, I was astounded to find that a large portion – about 1/3 – of what Google News returned was my work. And most of those articles had nothing to do with LGBTQ+ issues at all.
As I wrote then, we couldn’t have bought that kind of search domination. Had nobody else written about LGBTQ+ and AI all month? No, it was June so there were literally dozens of articles on the subject.
When I went to the Google Search site and performed the same query it produced different results. In fact, even if I clicked on the “news” tab from the main Search page, it still didn’t show the same ratio of one of my articles for every two from someone else.
The Google News interface, for whatever reason, seemingly draws information from my TNW author profile and uses it to influence query results.
Apparently there aren’t a lot of journalists who use the word “queer” in their indexed author page.
How it’s going
Heavy is the head that wears the crown and, to be honest with you, it can be quite taxing to be queer . I’d rather not be the queerest AI journo on the planet for more than a few weeks. So, a while back, I changed my author profile.
It used to say I covered “queer stuff,” because I thought that was kind of funny. I changed it to say I covered “LGTBTQ+ issues.” It seems more professional.
And, voila! Like magic, my reign was over. I searched for “artificial intelligence queer” on Google News and it returned a smaller number of my articles. And all of them were about being “queer” in STEM. Problem solved! Hooray?
No, of course not.
Admittedly, I’m no longer the queerest AI journo in the world . But hear me out because, as far as Google News is concerned now , my work on the “artificial intelligence LGBTQ+ issues” beat is important enough to take up even more of the search results than my work as a “queer” AI journalist.
Here we go again:
By changing my TNW author profile to say I covered “LGBTQ+ issues,” it turns out I was using a term that even fewer journalists use in their profiles.
If you search for “artificial intelligence LGBTQ+ issues” in Google News, about two- thirds of the articles in the search results are mine as of the time of this article’s publishing.
If I can’t escape my destiny as an ambassador between the queer AI journalist community and the Google News algorithm, I must embrace it.
The LGBTQ+iest journalist on the planet
And with great power comes great responsibility. Which is why I changed my TNW author profile to say I cover “Spiderman” today.
It could take a few days for the changes to reflect in Google indexes, but it’ll be worth the wait in my opinion.
If Google News is going to associate my articles with whatever I put in my author profile, I’m going to manifest a world where my name and Spiderman are synonymous. Why not?
The moral to this story is that algorithms are dumb. No human curator would have made the same mistakes.
And when algorithms deal with people, those mistakes can be harmful. Who knows how many journalists who wrote about technology for Pride this year lost page views because Google News decided to surface my articles about policy and physics instead of their relevant pieces?
Today, I’m able to exploit the algorithm for kicks. I can get my stories to show up under specific search queries in the Google News interface just by changing my author profile. It’s kind of funny.
But what if someone decides to silence queer journalism tomorrow by exploiting the algorithm in such a way that searching for news on LGBTQ+ issues, using any query string, would result in mostly Breitbart or Infowars articles surfacing? There’s nothing funny about that.
Update 6 January 2022: I’m honored to reveal to all of you that I am quantum computing Spiderman! Okay, so I couldn’t crack the algorithm and make it associate me with artificial intelligence and Spiderman. But, it did associate me with quantum computing and Spiderman, so I’ll count that as a win! As of the time of this update, if you search for “quantum computing spiderman” in the Google News app search bar, the overwhelming majority of results returned are from yours truly.
Related: Why can’t Google’s algorithms find any good news for queer people?
England will launch its digital vaccine passport next week
People in England will be able to use the National Health Service app as a vaccine passport from Monday, Health Secretary Matt Hancock confirmed today.
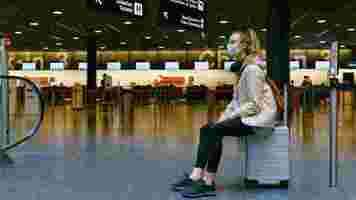
The digital certificates will be available from May 17, the same day that the country lifts its ban on non-essential travel. Holidaymakers who don’t have a smartphone will be able to request a paper version by calling the NHS helpline on 119.
“The certification, being able to show that you’ve had a jab, is going to be necessary for people to be able to travel,” Hancock told Sky News. “So, we want to make sure people can get access to that proof, not least to show governments of other countries that you’ve had the jab if they require that in order to arrive.”
The digital version will be displayed on the NHS app that was originally designed to book appointments and order repeat prescriptions, rather than the COVID-19 app that’s used for test and trace. To download the app, users need to be registered with a GP in England and aged 13 or over.
The app will initially only show the user’s vaccination record, although future updates will integrate their COVID-19 test results. The government says that vaccination status will be “held securely within the NHS App,” and only accessible via the NHS login service .
[ Read more : This dude drove an EV from the Netherlands to New Zealand — here are his 3 top road trip tips ]
The announcement will be welcomed by many holidaymakers hoping to travel abroad this summer, but their options remain limited for now.
“There are not many countries that currently accept proof of vaccination,” the government’s travel guidelines state. “So for the time being most people will still need to follow other rules when traveling abroad – like getting a negative pre-departure test.”
In addition, there are presently only 12 countries on the government’s green list of destinations that people can visit without quarantine on their return. However, Transport Secretary Grant Shapps said the restrictions will be reviewed every three weeks from May 17 “to see if we can expand the green list.”
Vaccine passports will initially be used for only international travel, but the government has previously suggested they may be later used for domestic venues such as pubs.
Civil liberties campaigners fear that approach will create a two-tier system in which some people can access freedoms and support that others are denied — with the most marginalized among us the hardest hit.
The Ada Lovelace Institute, a data ethics body, published a report yesterday that set out six requirements that governments must meet before permitting vaccine passports:
With the momentum for vaccine passports building, the institute hopes that setting high thresholds for their use will ensure that they benefit society.