New AI system counts endangered elephants from space
Scientists have unveiled a new tool for monitoring endangered wildlife: an AI system that automatically counts elephants from space.
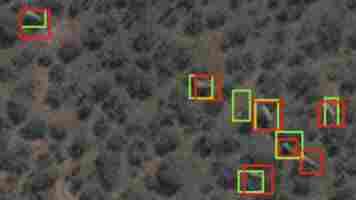
The tech combines satellite cameras with a convolutional neural network (CNN) to capture African elephants moving through forests and grasslands.
In tests, the surveying technique detected elephants as accurately as human observers, while eliminating the risk of disturbing the species.
The research joins a growing range of AI projects that are seeking to protect endangered animals .
“Accurate monitoring is essential if we’re to save the species,” said Dr Olga Isupova, a computer scientist at the University of Bath who created the detection algorithm. “We need to know where the animals are and how many there are.”
The system also offers a more efficient alternative to manually counting animals from low-flying airplanes. Each satellite can collect more than 5,000 km² of imagery every few minutes. And if clouds obscure the land, the process can be repeated the following day, on the satellite’s next revolution of Earth.
In addition, the technique can track animals as they roam across countries without having to worry about border controls or conflicts.
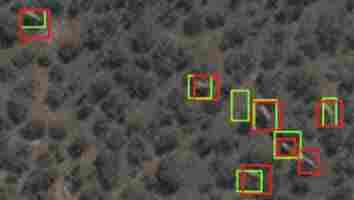
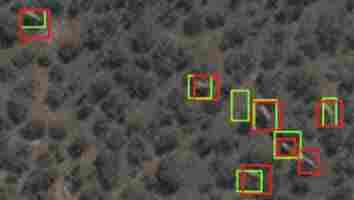
The researchers trained and tested their model on endangered African elephants in South Africa, using data from the WorldView‐3 and WorldView‐4 satellites — the highest resolution satellite imagery that’s commercially available.
They also applied the model to lower resolution satellite images captured in Kenya — without any additional training data — to test whether it could work outside their study area.
“Our results show that the CNN performs with high accuracy, comparable to human detection capabilities,” the team wrote in their research paper.
They chose African elephants for the study because they’re the largest land animal in the world — which makes them relatively easy to spot. But the team believes their system could soon detect far smaller species.
“Satellite imagery resolution increases every couple of years, and with every increase, we will be able to see smaller things in greater detail,” said Dr Isupova.
You can read the study paper in the journal Remote Sensing in Ecology and Conservation .
Human intuition and machine reasoning: AI’s helping us solve giant math puzzles
Research in mathematics is a deeply imaginative and intuitive process. This might come as a surprise for those who are still recovering from high-school algebra.
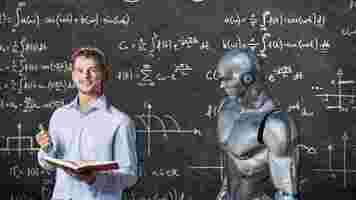
What does the world look like at the quantum scale? What shape would our universe take if we were as large as a galaxy? What would it be like to live in six or even 60 dimensions? These are the problems that mathematicians and physicists are grappling with every day.
To find the answers, mathematicians like me try to find patterns that relate complicated mathematical objects by making conjectures (ideas about how those patterns might work), which are promoted to theorems if we can prove they are true. This process relies on our intuition as much as our knowledge.
Over the past few years I’ve been working with experts at artificial intelligence (AI) company DeepMind to find out whether their programs can help with the creative or intuitive aspects of mathematical research. In a new paper published in Nature , we show they can: recent techniques in AI have been essential to the discovery of a new conjecture and a new theorem in two fields called “knot theory” and “representation theory”.
Machine intuition
Where does the intuition of a mathematician come from? One can ask the same question in any field of human endeavour. How does a chess grandmaster know their opponent is in trouble? How does a surfer know where to wait for a wave?
The short answer is we don’t know. Something miraculous seems to happen in the human brain. Moreover, this “miraculous something” takes thousands of hours to develop and is not easily taught.
The past decade has seen computers display the first hints of something like human intuition. The most striking example of this occurred in 2016, in a Go match between DeepMind’s AlphaGo program and Lee Sedol, one of the world’s best players.
AlphaGo won 4–1, and experts observed that some of AlphaGo’s moves displayed human-level intuition. One particular move ( “move 37” ) is now famous as a new discovery in the game.
How do computers learn?
Behind these breakthroughs lies a technique called deep learning. On a computer one builds a neural network – essentially a crude mathematical model of a brain, with many interconnected neurons.
At first, the network’s output is useless. But over time (from hours to even weeks or months), the network is trained, essentially by adjusting the firing rates of the neurons.
Such ideas were tried in the 1970s with unconvincing results. Around 2010, however, a revolution occurred when researchers drastically increased the number of neurons in the model (from hundreds in the 1970s to billions today).
Traditional computer programs struggle with many tasks humans find easy, such as natural language processing (reading and interpreting text), and speech and image recognition.
With the deep learning revolution of the 2010s, computers began performing well on these tasks. AI has essentially brought vision and speech to machines.
Training neural nets requires huge amounts of data. What’s more, trained deep learning models often function as “black boxes”. We know they often give the right answer, but we usually don’t know (and can’t ascertain) why.
A lucky encounter
My involvement with AI began in 2018, when I was elected a Fellow of the Royal Society. At the induction ceremony in London I met Demis Hassabis, chief executive of DeepMind.
Over a coffee break we discussed deep learning, and possible applications in mathematics. Could machine learning lead to discoveries in mathematics, like it had in Go?
This fortuitous conversation led to my collaboration with the team at DeepMind.
Mathematicians like myself often use computers to check or perform long computations. However, computers usually cannot help me develop intuition or suggest a possible line of attack. So we asked ourselves: can deep learning help mathematicians build intuition?
With the team from DeepMind, we trained models to predict certain quantities called Kazhdan-Lusztig polynomials, which I have spent most of my mathematical life studying.
In my field we study representations , which you can think of as being like molecules in chemistry. In much the same way that molecules are made of atoms, the make up of representations is governed by Kazhdan-Lusztig polynomials.
Amazingly, the computer was able to predict these Kazhdan-Lusztig polynomials with incredible accuracy. The model seemed to be onto something, but we couldn’t tell what.
However, by “peeking under the hood” of the model, we were able to find a clue which led us to a new conjecture: that Kazhdan-Lusztig polynomials can be distilled from a much simpler object (a mathematical graph).
This conjecture suggests a way forward on a problem that has stumped mathematicians for more than 40 years. Remarkably, for me, the model was providing intuition!
In parallel work with DeepMind, mathematicians Andras Juhasz and Marc Lackenby at the University of Oxford used similar techniques to discover a new theorem in the mathematical field of knot theory. The theorem gives a relation between traits (or “invariants”) of knots that arise from different areas of the mathematical universe.
Our paper reminds us that intelligence is not a single variable, like the result of an IQ test. Intelligence is best thought of as having many dimensions.
My hope is that AI can provide another dimension, deepening our understanding of the mathematical world, as well as the world in which we live.
This article by Geordie Williamson , Professor of Mathematics, University of Sydney , is republished from The Conversation under a Creative Commons license. Read the original article .
Will AI coding assistants like GitHub’s Copilot transform developers’ jobs?
OpenAI has once again made the headlines, this time with Copilot, an AI-powered programming tool jointly built with GitHub. Built on top of GPT-3 , OpenAI’s famous language model, Copilot is an autocomplete tool that provides relevant (and sometimes lengthy) suggestions as you write code.
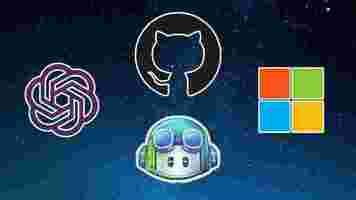
Copilot is currently available to select applicants as an extension in Visual Studio Code, the flagship programming tool of Microsoft, GitHub’s parent company.
While the AI-powered code generator is still a work in progress, it provides some interesting hints about the business of large language models and the future directions of the software industry.
Not the intended use for GPT-3
The official website of Copilot describes it as an “AI pair programmer” that suggests “whole lines or entire functions right inside your editor.” Sometimes, just providing a function signature or description is enough to generate an entire block of code.
Working behind Copilot is a deep learning model called Codex, which is basically a special version of GPT-3 finetuned for programming tasks. The tool’s working is very much like GPT-3: It takes a prompt as input and generates a sequence of bytes as output. Here, the prompt (or context) is the source code file you’re working on and the output is the code suggestion you receive.
What’s interesting in all of this is the unexpected turns AI product management can take. According to CNBC : “…back when OpenAI was first training [GPT-3], the start-up had no intention of teaching it how to help code, [OpenAI CTO Greg] Brockman said. It was meant more as a general purpose language model [emphasis mine] that could, for instance, generate articles, fix incorrect grammar and translate from one language into another.”
General-purpose language applications have proven to be very hard to nail . There are many intricacies involved when applying natural language processing to broad environments. Humans tend to use a lot of abstractions and shortcuts in day-to-day language. The meaning of words, phrases, and sentences can vary based on shared sensory experience, work environment, prior knowledge, etc. These nuances are hard to grasp with deep learning models that have been trained to grasp the statistical regularities of a very large dataset of anything and everything.
In contrast, language models perform well when they’re provided with the right context and their application is narrowed down to a single or a few related tasks. For example, deep learning–powered chatbots trained or finetuned on a large corpus of customer chats can be a decent complement to customer service agents, taking on the bulk of simple interactions with customers and leaving complicated requests to human operators. There are already plenty of special-purpose deep learning models for different language tasks.
Therefore, it’s not very surprising that the first applications for GPT-3 have been something other than general-purpose language tasks.
Using language models for coding
Shortly after GPT-3 was made available through a beta web application programming interface, many users posted examples of using the language model to generate source code. These experiments displayed an unexplored side of GPT-3 and a potential use case for the large language model.
And interestingly, the first two applications that Microsoft, the exclusive license holder of OpenAI’s language models, created on top of GPT-3 are related to computer programming. In May, Microsoft announced a GPT-3-powered tool that generates queries for its Power Apps. And now, it is testing the waters with Copilot.
Neural networks are very good at finding and suggesting patterns from large training datasets. In this light, it makes sense to use GPT-3 or a finetuned version of it to help programmers find solutions in the very large corpus of publicly available source code in GitHub.
According to Copilot’s homepage , OpenAI Codex, which powers Copilot, has been trained on “a selection of English language and source code from publicly available sources, including code in public repositories on GitHub.”
If you provide it with the right context, it will be able to come up with a block of code that resembles what other programmers have written to solve a similar problem. And giving it more detailed comments and descriptions will improve your chances of getting a reasonable output from Copilot.
Generating code vs understanding software
According to the website, “GitHub Copilot tries to understand [emphasis mine] your intent and to generate the best code it can, but the code it suggests may not always work, or even make sense.”
“Understand” might be the wrong word here . Language models such as GPT-3 do not understand the purpose and structure of source code. They don’t understand the purpose of programs. They can’t come up with new ideas, break down a problem into smaller components, and design and build an application in the way that human software engineers do.
By human standards, programming is a relatively difficult task (well, it used to be when I was learning in the 90s). It requires careful thinking, logic, and architecture design to solve a specific problem. Each language has its own paradigms and programming patterns. Developers must learn to use different application programming interfaces and plug them together in an efficient way. In short, it’s a skill that is largely dependent on symbol manipulation , an area that is not the forte of deep learning algorithms.
Copilot’s creators acknowledge that their AI system is in no way a perfect programming companion (I don’t even think “ pair programming ,” is the right term for it). “GitHub Copilot doesn’t actually test the code it suggests, so the code may not even compile or run,” they warn.
GitHub also warns that Copilot may suggest “old or deprecated uses of libraries and languages,” which can cause security issues. This makes it extremely important for developers to review the AI-generated code thoroughly.
So, we’re not at a stage to expect AI systems to automate programming. But pairing them with humans who know what they’re doing can surely improve productivity, as Copilot’s creators suggest.
And since Copilot was released to the public, developers have posted all kinds of examples ranging from amusing to really useful.
“If you know a bit about what you’re asking Copilot to code for you, and you have enough experience to clean up the code and fix the errors that it introduces, it can be very useful and save you time,” Matt Shumer, co-founder and CEO of OthersideAI , told TechTalks.
But Shumer also warns about the threats of blindly trusting the code generated by Copilot.
“For example, it saved me time writing SQL code, but it put the database password directly in the code,” Shumer said. “If I wasn’t experienced, I might accept that and leave it in the code, which would create security issues. But because I knew how to modify the code, I was able to use what Copilot gave me as a starting point to work off of.”
The business model of Copilot
In my opinion, there’s another reason for which Microsoft started out with programming as the first application for GPT-3. There’s a huge opportunity to cut costs and make profits.
According to GitHub, “If the technical preview is successful, our plan is to build a commercial version of GitHub Copilot in the future.”
There’s still no information on how much the official Copilot will cost. But hourly wages for programming talent start at around $30 and can reach as high as $150. Even saving a few hours of programming time or giving a small boost to development speed would probably be enough to cover the costs of Copilot. Therefore, it would not be surprising if many developers and software development companies would sign up for Copilot once it is released as a commercial product.
“If it gives me back even 10 percent of my time, I’d say it’s worth the cost. Within reason, of course,” Shumer said.
Language models like GPT-3 require extensive resources to train and run . And they also need to be regularly updated and finetuned, which imposes more expenses on the company hosting the machine learning model. Therefore, high-cost domains such as software development would be a good place to start to reduce the time to recoup the investment made on the technology.
“The ability for [Copilot] to help me use libraries and frameworks I’ve never used before is extremely valuable,” Shumer said. “In one of my demos, for example, I asked it to generate a dashboard with Streamlit, and it did it perfectly in one try. I could then go and modify that dashboard, without needing to read through any documentation. That alone is valuable enough for me to pay for it.”
Automated coding can turn out to be a multi-billion-dollar industry. And Microsoft is positioning itself to take a leading role in this nascent sector , thanks to its market reach (through Visual Studio, Azure, and GitHub), deep pockets, and exclusive access to OpenAI’s technology and talent.
The future of automated coding
Developers must be careful not to mistake Copilot and other AI-powered code generators for a programming companion whose every suggestion you accept. As a programmer who has worked under tight deadlines on several occasions, I know that developers tend to cut corners when they’re running out of time (I’ve done it more than a few times). And if you have a tool that gives you a big chunk of working code in one fell swoop, you’re prone to just skim over it if you’re short on time.
On the other hand, adversaries might find ways to track vulnerable coding patterns in deep learning code generators and find new attack vectors against AI-generated software .
New coding tools create new habits (many of them negative and insecure). We must carefully explore this new space and beware the possible tradeoffs of having AI agents as our new coding partners.
This article was originally published by Ben Dickson on TechTalks , a publication that examines trends in technology, how they affect the way we live and do business, and the problems they solve. But we also discuss the evil side of technology, the darker implications of new tech, and what we need to look out for. You can read the original article here .