Qualcomm’s betting on AI to take on Apple and Google’s chips
Apple has been in a league of its own when it comes to developing processors for phones and embedding its own AI-powered experience.
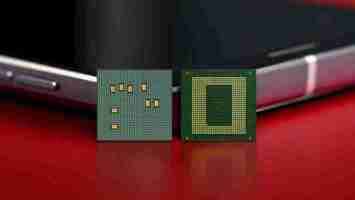
But this year, Google has thrown its hat in the ring by debuting its indigenous Tensor Chip to give the Pixel 6 a platform to showcase the company’s vision for smartphones.
This massive push by tech giants into the chip game means that Qualcomm, once a leading player in the smartphone industry , will face more competition than ever. So, this year, the chipmaker is relying heavily on AI to give its new processor, the Snapdragon 8 Gen 1, a bit more personality.
The company is introducing the 7th Gen Qualcomm AI compute engine, which is four times faster than its predecessor.
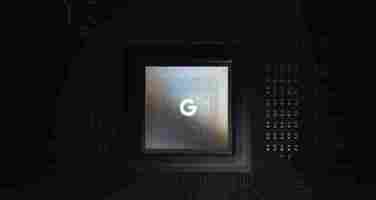
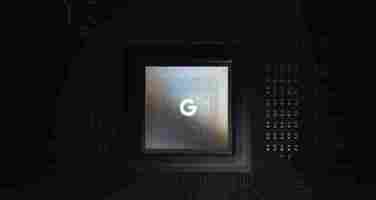
The chipmaker claims that the new unit is more powerful because it gets double the shared memory and a twice-as-fast Tensor accelerator for AI-related operations. Plus, the battery efficiency is 2.8 times better than this year’s flagship Snapdragon 888 processor.
And in addition to that, there’s a new 3rd-gen sensing unit that can enable ultra low-powered voice and connectivity experiences.
So now that we’ve seen the new chip’s AI specs, let’s take a look at what it can mean for phone makers, developers, and consumers.
What can the new AI unit do?
Vinesh Sukumar, Head of AI/ML Product Management at Qualcomm, said the new AI unit can handle a lot more workload easily. The company developed a demo with video imaging company Arcsoft, where a prototype phone based on the Snapdragon 8 Gen 1 could simultaneously perform face detection and apply the face bokeh effect.
Sukumar said that the new tech allows the software to detect more than 20 points on the face, so app makers can apply effects even when there’s a bad angle or small field of view.
The new sensing hub enables the chip to have always-on camera experiences for face and object detection while consuming low power.
There’s also a new ‘always secure’ module within the sensing hub that allows apps to conduct financial transactions in a safer way.
Why is Qualcomm partnering with companies for different experiences?
Until now, Qualcomm chips usually provided phone makers with a canvas to draw their own paintings. While that still might be the case, this year, the company is partnering with many firms to give different AI-powered experiences a bit more flair.
First, for an enhanced camera experience, including charming black and white shots and bokeh effects, Qualcomm is partnering with the German imaging company Leica. It has previously worked with manufacturers such as Huawei and Xiaomi . But this is a rare occasion when a chipmaker is working with a camera maker exclusively.
Sukumar said camera maker Leica was a key partner in developing AI to capture high-quality and dense images with great spatial details. He said that this tech will enhance dynamic range and clarity in pictures.
Qualcomm also partnered with health organization Sonde to showcase an app that records your voice for 30 seconds and detects your stress levels, asthma condition, and even COVID-19.
This is not entirely novel: Israeli startup Vocalis has achieved a similar feat with an app , but in Qualcomm’s case, all the processing is done locally to keep the user privacy intact.
One of the most important partnerships is with Hugging Face , an open-source startup working on language-based machine learning models. Qualcomm demoed a smart assistant which uses sentiment analysis to classify the most important notifications for you. Plus, you can look at a summary of notifications based on their priority.
The assistant can also answer questions like “Where’s my dinner meeting?” and “When should I leave?”
All this reminded me of Google Assistant. But Qualcomm’s Vice President and Head of AI Software, Jeff Gehlhaar, told me that this partnership will bring a broader portfolio of use cases. I’d be very interested to see an assistant that has better capabilities than Google Assistant, so bring it on Qualcomm.
What’s in it for developers?
Qualcomm said this year it’s introducing support for mixed-precision instructions in its SDK, so developers can make their AI models run faster while using less memory.
However, the biggest change is to introduce Neural architecture search (NAS) for Qualcomm chips. NAS enables data scientists to optimize a model for particular hardware without manually training it. They can also put constraints on the model’s size or other parameters based on the use case.
The chipmaker is partnering with Google Cloud to provide the backbone for this NAS offering. Qualcomm will use t he Google Vertex AI solution for managed machine learning, so developers can train their models for its chips easily.
This partnership will allow developers to tune their models not just for mobile, but for Qualcomm’s XR, IoT, and automotive solutions as well.
A battle with Apple, Samsung, and Google
Apple always had its own chips on its iPhones, while Samsung uses a mix of its indigenous Exynos processors and Qualcomm’s Snapdragon processors for its devices.
This year, Google ditched Qualcomm for the first time, so the competition in the chip market is definitely heating up.
The US-based chip giant will need to show its processors are as capable as Apple’s, Samsung’s, or Google’s, if not better. And for that, it’s putting the onus on its AI chops.
It’ll be amazing to see companies like OnePlus, Samsung, or Xiaomi using Qualcomm’s new gear to bring out an AI experience that we’ve not seen on iPhones or Pixels. Game on!
What is semi-supervised machine learning?
Machine learning has proven to be very efficient at classifying images and other unstructured data, a task that is very difficult to handle with classic rule-based software . But before machine learning models can perform classification tasks, they need to be trained on a lot of annotated examples. Data annotation is a slow and manual process that requires humans to review training examples one by one and giving them their right labels.
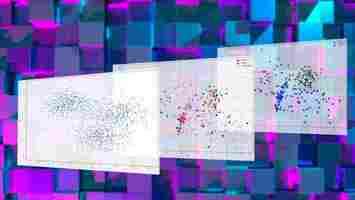
In fact, data annotation is such a vital part of machine learning that the growing popularity of the technology has given rise to a huge market for labeled data. From Amazon’s Mechanical Turk to startups such as LabelBox, ScaleAI, and Samasource, there are dozens of platforms and companies whose job is to annotate data to train machine learning systems.
Fortunately, for some classification tasks, you don’t need to label all your training examples. Instead, you can use semi-supervised learning, a machine learning technique that can automate the data-labeling process with a bit of help.
Supervised vs unsupervised vs semi-supervised machine learning
You only need labeled examples for supervised machine learning tasks, where you must specify the ground truth for your AI model during training. Examples of supervised learning tasks include image classification, facial recognition, sales forecasting, customer churn prediction, and spam detection .
Unsupervised learning, on the other hand, deals with situations where you don’t know the ground truth and want to use machine learning models to find relevant patterns. Examples of unsupervised learning include customer segmentation , anomaly detection in network traffic, and content recommendation.
Semi-supervised learning stands somewhere between the two. It solves classification problems, which means you’ll ultimately need a supervised learning algorithm for the task. But at the same time, you want to train your model without labeling every single training example, for which you’ll get help from unsupervised machine learning techniques.
Semi-supervised learning with clustering and classification algorithms
One way to do semi-supervised learning is to combine clustering and classification algorithms. Clustering algorithms are unsupervised machine learning techniques that group data together based on their similarities. The clustering model will help us find the most relevant samples in our data set. We can then label those and use them to train our supervised machine learning model for the classification task.
Say we want to train a machine learning model to classify handwritten digits, but all we have is a large data set of unlabeled images of digits. Annotating every example is out of the question and we want to use semi-supervised learning to create your AI model.
First, we use k-means clustering to group our samples. K-means is a fast and efficient unsupervised learning algorithm, which means it doesn’t require any labels. K-means calculates the similarity between our samples by measuring the distance between their features. In the case of our handwritten digits, every pixel will be considered a feature, so a 20×20-pixel image will be composed of 400 features.
When training the k-means model, you must specify how many clusters you want to divide your data into. Naturally, since we’re dealing with digits, our first impulse might be to choose ten clusters for our model. But bear in mind that some digits can be drawn in different ways. For instance, here are different ways you can draw the digits 4, 7, and 2. You can also think of various ways to draw 1, 3, and 9.
Therefore, in general, the number of clusters you choose for the k-means machine learning model should be greater than the number of classes. In our case, we’ll choose 50 clusters, which should be enough to cover different ways digits are drawn.
After training the k-means model, our data will be divided into 50 clusters. Each cluster in a k-means model has a centroid , a set of values that represent the average of all features in that cluster. We choose the most representative image in each cluster, which happens to be the one closest to the centroid. This leaves us with 50 images of handwritten digits.
Now, we can label these 50 images and use them to train our second machine learning model, the classifier, which can be a logistic regression model, an artificial neural network , a support vector machine, a decision tree, or any other kind of supervised learning engine.
Training a machine learning model on 50 examples instead of thousands of images might sound like a terrible idea. But since the k-means model chose the 50 images that were most representative of the distributions of our training data set, the result of the machine learning model will be remarkable. In fact, the above example, which was adapted from the excellent book Hands-on Machine Learning with Scikit-Learn, Keras, and Tensorflow , shows that training a regression model on only 50 samples selected by the clustering algorithm results in a 92-percent accuracy (you can find the implementation in Python in this Jupyter Notebook ). In contrast, training the model on 50 randomly selected samples results in 80-85-percent accuracy.
But we can still get more out of our semi-supervised learning system. After we label the representative samples of each cluster, we can propagate the same label to other samples in the same cluster. Using this method, we can annotate thousands of training examples with a few lines of code. This will further improve the performance of our machine learning model.
Other semi-supervised machine learning techniques
There are other ways to do semi-supervised learning, including semi-supervised support vector machines (S3VM), a technique introduced at the 1998 NIPS conference. S3VM is a complicated technique and beyond the scope of this article. But the general idea is simple and not very different from what we just saw: You have a training data set composed of labeled and unlabeled samples. S3VM uses the information from the labeled data set to calculate the class of the unlabeled data, and then uses this new information to further refine the training data set.
If you’re are interested in semi-supervised support vector machines, see the original paper and read Chapter 7 of Machine Learning Algorithms , which explores different variations of support vector machines (an implementation of S3VM in Python can be found here ).
An alternative approach is to train a machine learning model on the labeled portion of your data set, then using the same model to generate labels for the unlabeled portion of your data set. You can then use the complete data set to train an new model.
The limits of semi-supervised machine learning
Semi-supervised learning is not applicable to all supervised learning tasks. As in the case of the handwritten digits, your classes should be able to be separated through clustering techniques. Alternatively, as in S3VM, you must have enough labeled examples, and those examples must cover a fair represent the data generation process of the problem space.
But when the problem is complicated and your labeled data are not representative of the entire distribution, semi-supervised learning will not help. For instance, if you want to classify color images of objects that look different from various angles, then semi-supervised learning might help much unless you have a good deal of labeled data (but if you already have a large volume of labeled data, then why use semi-supervised learning?). Unfortunately, many real-world applications fall in the latter category, which is why data labeling jobs won’t go away any time soon.
But semi-supervised learning still has plenty of uses in areas such as simple image classification and document classification tasks where automating the data-labeling process is possible.
Semi-supervised learning is a brilliant technique that can come handy if you know when to use it.
This article was originally published by Ben Dickson on TechTalks , a publication that examines trends in technology, how they affect the way we live and do business, and the problems they solve. But we also discuss the evil side of technology, the darker implications of new tech and what we need to look out for. You can read the original article here .
New AI from DeepMind and Google can detect a common cause of blindness
DeepMind and Google Health have developed an AI that can predict who’ll get one of the world’s most common causes of blindness.
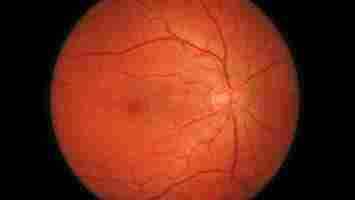
The system was built to detect age-related macular degeneration (AMD), a disease that afflicts more than 25% of over-60s in Europe and up to 11 million people in the US .
There are two types of the disease: a “dry” form, which often only causes mild sight loss, and a “wet” one, which can lead to permanent blindness.
Currently, Ophthalmologists diagnose AMD by analyzing 3D scans of the eye. But these highly detailed images are time-consuming to review. DeepMind‘s researchers suspected that AI could more quickly detect the symptoms of patients needing urgent treatment – which could ultimately save their sight.
Developing the model
The team trained their model on an anonymized dataset of retinal scans from 2,795 patients who had been diagnosed with wet AMD.
They then used two neural networks to detect the disease by analyzing 3D eye scans and labeling features that offered clues about the diseases. The system uses this data to estimate whether the patient would develop wet ADM within the next six months.
DeepMind claims that the system predicted the onset of wet AMD as well as human experts — and in some cases was even more accurate.
Pearse Keane, an ophthalmologist who worked on the project, said the system could act as an early warning sign for the disease:
The next step is validating the algorithm so it can be used in clinical trials. In time, it could even help doctors develop treatments for the disease.