Self-driving startup Waabi just managed to net $83.5M — how?
It is not the best of times for self-driving car startups. The past year has seen large tech companies acquire startups that were running out of cash and ride-hailing companies shutter costly self-driving car projects with no prospect of becoming production-ready anytime soon.
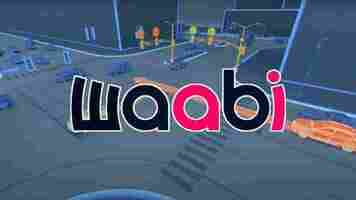
Yet, in the midst of this downturn, Waabi, a Toronto-based self-driving car startup, has just come out of stealth with an insane amount of $83.5 million in a Series A funding round led by Khosla Ventures, with additional participation from Uber, 8VC, Radical Ventures, OMERS Ventures, BDC, and Aurora Innovation. The company’s financial backers also include Geoffrey Hinton , Fei-Fei Li, Peter Abbeel, and Sanja Fidler, artificial intelligence scientists with great influence in the academia and applied AI community.
What makes Waabi qualified for such support? According to the company’s press release , Waabi aims to solve the “scale” challenge of self-driving car research and “bring commercially viable self-driving technology to society.” Those are two key challenges of the self-driving car industry and are mentioned numerous times in the release.
What Waabi describes as its “next generation of self-driving technology” has yet to pass the test of time. But its execution plan provides hints at what directions the self-driving car industry could be headed.
Better machine learning algorithms and simulations
According to Waabi’s press release: “The traditional approach to engineering self-driving vehicles results in a software stack that does not take full advantage of the power of AI, and that requires complex and time-consuming manual tuning. This makes scaling costly and technically challenging, especially when it comes to solving for less frequent and more unpredictable driving scenarios.”
Leading self-driving car companies have driven their cars on real roads for millions of miles to train their deep learning models . Real-road training is costly both in terms of logistics and human resources. It is also fraught with legal challenges as the laws surrounding self-driving car tests vary in different jurisdictions. Yet despite all the training, self-driving car technology struggles to handle corner cases, rare situations that are not included in the training data. These mounting challenges speak to the limits of current self-driving car technology.
Here’s how Waabi claims to solve these challenges (emphasis mine): “The company’s breakthrough, AI-first approach , developed by a team of world leading technologists, leverages deep learning, probabilistic inference and complex optimization to create software that is end-to-end trainable, interpretable and capable of very complex reasoning . This, together with a revolutionary closed loop simulator that has an unprecedented level of fidelity , enables testing at scale of both common driving scenarios and safety-critical edge cases . This approach significantly reduces the need to drive testing miles in the real world and results in a safer, more affordable, solution.”
There’s a lot of jargon in there (a lot of which is probably marketing lingo) that needs to be clarified. I reached out to Waabi for more details and will update this post if I hear back from them.
By “AI-first approach,” I suppose they mean that they will put more emphasis on creating better machine learning models and less on complementary technology such as lidars, radars, and mapping data. The benefit of having a software-heavy stack is the very low costs of updating the technology. And there will be a lot of updating in the coming years as scientists continue to find ways to circumvent the limits of self-driving AI .
The combination of “deep learning, probabilistic reasoning, and complex optimization” is interesting, albeit not a breakthrough. Most deep learning systems use non-probabilistic inference. They provide an output, say a category or a predicted value, without giving the level of uncertainty on the result. Probabilistic deep learning, on the other hand, also provides the reliability of its inferences, which can be very useful in critical applications such as driving.
“End-to-end trainable” machine learning models require no manual-engineered features. This means once you have developed the architecture and determined the loss and optimization functions, all you need to do is provide the machine learning model with training examples. Most deep learning models are end-to-end trainable. Some of the more complicated architectures require a combination of hand-engineered features and knowledge along with trainable components.
Finally, “ interpretability ” and “ reasoning ” are two of the key challenges of deep learning. Deep neural networks are composed of millions and billions of parameters. This makes it hard to troubleshoot them when something goes wrong (or find problems before something bad happens), which can be a real challenge in critical scenarios such as driving cars. On the other hand, the lack of reasoning power and causal understanding makes it very difficult for deep learning models to handle situations they haven’t seen before.
According to TechCrunch’s coverage of Waabi’s launch, Raquel Urtasun, the company’s CEO, described the AI system the company uses as a “family of algorithms.”
“When combined, the developer can trace back the decision process of the AI system and incorporate prior knowledge so they don’t have to teach the AI system everything from scratch,” TechCrunch wrote.
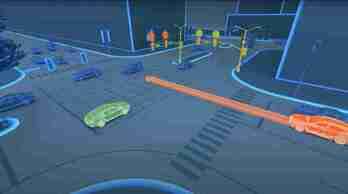
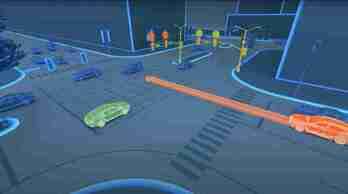
The closed-loop simulation environment is a replacement for sending real cars on real roads. In an interview with The Verge , Urtasun said that Waabi can “test the entire system” in simulation. “We can train an entire system to learn in simulation, and we can produce the simulations with an incredible level of fidelity, such that we can really correlate what happens in simulation with what is happening in the real world.”
I’m a bit on the fence on the simulation component. Most self-driving car companies are using simulations as part of the training regime of their deep learning models. But creating simulation environments that are exact replications of the real world is virtually impossible, which is why self-driving car companies continue to use heavy road testing.
Waymo has at least 20 billion miles of simulated driving to go with its 20 million miles of real-road testing, which is a record in the industry. And I’m not sure how a startup with $83.5 million in funding can outmatch the talent, data, compute, and financial resources of a self-driving company with more than a decade of history and the backing of Alphabet, one of the wealthiest companies in the world.
More hints of the system can be found in the work that Urtasun, who is also a professor in the Department of Computer Science at the University of Toronto, does in academic research. Urtasan’s name appears on many papers about autonomous driving. But one, in particular, uploaded on the arXiv preprint server in January, is interesting.
Titled, “ MP3: A Unified Model to Map, Perceive, Predict and Plan ,” the paper discusses an approach to self-driving that is very close to the description in Waabi’s launch press release.
The researchers describe MP3 as “an end-to-end approach to mapless driving that is interpretable, does not incur any information loss, and reasons about uncertainty in the intermediate representations.” In the paper researchers also discuss the use of “probabilistic spatial layers to model the static and dynamic parts of the environment.”
MP3 is end-to-end trainable and uses lidar input to create scene representations, predict future states, and plan trajectories. The machine learning model obviates the need for finely detailed mapping data that companies like Waymo use in their self-driving vehicles.
Urtasun posted a video on her YouTube that provides a brief explanation of how MP3 works. It’s fascinating work, though many researchers will point out that it not so much of a breakthrough as a clever combination of existing techniques.
There’s also a sizeable gap between academic AI research and applied AI. It remains to be seen if MP3 or a variation of it is the model that Waabi is using and how it will perform in practical settings.
A more conservative approach to commercialization
Waabi’s first application will not be passenger cars that you can order with your Lyft or Uber app.
“The team will initially focus on deploying Waabi’s software in logistics, specifically long-haul trucking, an industry where self-driving technology stands to make the biggest and swiftest impact due to a chronic driver shortage and pervasive safety issues,” Waabi’s press release states.
What the release doesn’t mention, however, is that highway settings are an easier problem to solve because they are much more predictable than urban areas. This makes them less prone to edge cases (such as a pedestrian running in front of the car) and easier to simulate. Self-driving trucks can transport cargo between cities, while human drivers take care of delivery inside cities.
With Lyft and Uber failing to launch their own robo-taxi services, and with Waymo still away from turning One, its fully driverless ride-hailing service, into a scalable and profitable business , Waabi’s approach seems to be well thought.
With more complex applications still being beyond reach, we can expect self-driving technology to make inroads into more specialized settings such as trucking and industrial complexes and factories .
Waabi also doesn’t make any mention of a timeline in the press release. This also seems to reflect the failures of the self-driving car industry in the past few years. Top executives of automotive and self-driving car companies have constantly made bold statements and given deadlines about the delivery of fully driverless technology . None of those deadlines have been met.
Whether Waabi becomes independently successful or ends up joining the acquisition portfolio of one of the tech giants, its plan seems to be a reality check on the self-driving car industry. The industry needs companies that can develop and test new technologies without much fanfare, embrace change as they learn from their mistakes, make incremental improvements, and save their cash for a long race.
This article was originally published by Ben Dickson on TechTalks , a publication that examines trends in technology, how they affect the way we live and do business, and the problems they solve. But we also discuss the evil side of technology, the darker implications of new tech, and what we need to look out for. You can read the original article here.
Ford and Google buddy up to bring Android to ‘millions’ of cars from… 2023
American automotive giant Ford has inked a deal with Google that will bring Android Automotive to “ millions ” of cars.
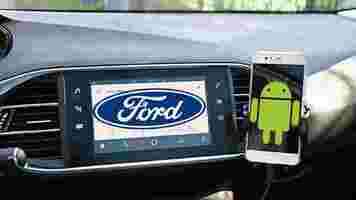
In a Medium post yesterday , Ford announced that it’s partnering with the Big G to help modernize its plants through use of AI, use its cloud services, develop new retail experiences, and create new ownership offers using connected vehicle data.
It also means that Ford will use Android Automotive as the base operating system for its vehicles from 2023, and that’s big, big news.
Why? Ford sells millions of vehicles each year. It’s as simple as that. In the US alone in 2019, the company sold 2.4 million vehicles . Even though it won’t come until 2023, it will put Android Auto at the fingertips of millions of drivers around the world.
Thanks to Android’s open source nature, with millions of cars running the software, it all of a sudden becomes a valuable opportunity for independent developers. It will no doubt lead to many exciting apps, in a totally new context.
Ford hasn’t said what models will get Android Automotive, though. Only that “millions” of Ford and Lincoln cars will.
The American automaker isn’t going full Google on its infotainment system, though. Ford is going to retain a number of its key features, such as its Sync user interface and operating system, which will just be built on top of Android rather than Blackberry QNX, the company’s current system supplier.
The deal makes Ford the latest to join a small, but growing, group of carmakers that are getting on board with Android for their cars. Android Automotive first debuted in the Polestar 2 last year, and will be seen in vehicles from General Motors later this year.
Folks at Google are no doubt swinging from the rafters with joy over this announcement, as it’s going to get its product at the hands of so many people.
It’s also great news for drivers, if you ask me. Having used Android Automotive in the Polestar 2, its voice recognition blew me away, it was simple and intuitive to use, and everything just made sense, because it’s just like using an Android phone, but in a car.
Even these days, infotainment systems can be hit or miss. Google can do software, so let’s have at it. Fords with Android Automotive can’t come soon enough.
SHIFT is brought to you by Polestar. It’s time to accelerate the shift to sustainable mobility. That is why Polestar combines electric driving with cutting-edge design and thrilling performance. Find out how .
Is fast charging bad for your EV battery?
When fast chargers came to town, they solved a huge EV issue: long charging times. After all, who’d prefer to wait for hours instead of juicing up their car within 20 to 30 minutes?
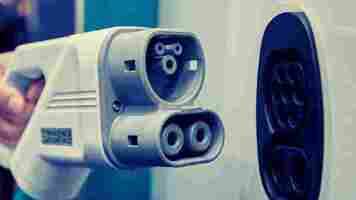
But, there’s one thing we need to keep in mind: we shouldn’t overdo it. DC fast chargers (or Level 3) degrade the battery faster than AC chargers (or Level 1 and 2) do.
Rapidly charging a battery means that high currents are created that result in high temperatures — and both are known to strain batteries.
Studies reveal there is indeed a noticeable deterioration rate.
According to Geotab , for instance, the battery’s state of health (SOH) is affected by how often a vehicle is using DC fast charging.
The telematics company specifically examined EVs operating in hot weather conditions that have proven to be more straining for the battery.
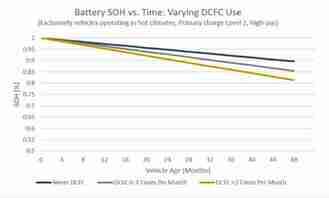
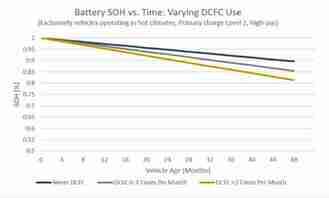
Battery degradation appears to be strongly correlated with the use of DC chargers for vehicles in seasonal or hot climates.
Compared to EVs that don’t use fast charging at all, vehicles that primarily do use it show a battery deterioration by nearly 0.1%.
The graph below compares how Level 1 and Level 2 (AC) chargers affect the battery’s state of health:
It’s evident that Level 2 charging has the least negative impact, reaching 0.9%. What’s more, both Level 1 and Level 2 charging damage the battery less than the frequent use of DC chargers.
We can observe, however, that the difference between Level 2 and Level 3 isn’t that big: approximately 0.1%.
The Idaho National Laboratory (INL) has made similar findings . The US Energy Department lab concluded that while an electric car’s battery will deteriorate faster if its only power source is Level 3 charging (which is almost never the case), the difference isn’t particularly pronounced.
The INL tested two pairs of the 2012 Nissan Leaf under the exact same conditions over the course of a year, in Phoenix, Arizona.
The first pair was charged with Level 2 chargers, and the second pair with Level 3 fast chargers. Both pairs were charged twice a day.
After all four test cars had been driven for 80,467 kilometers, the Level 2 vehicles had lost around 23% of their original battery capacity, while the Level 3 cars were down by around 27%.
Fast charging deteriorates the battery faster than Level 2 charging, but that’s not necessarily worrisome for your EV, providing that DC chargers aren’t the primary source.
Still, prolonging a battery’s life is a pretty big deal given its cost, so there are a few things that we can do even if we enjoy the convenience of fast charging: