The AGI hype train is running out of steam
The AGI hype train has hit some heavy traffic.
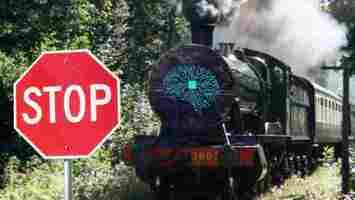
While futurists and fundraisers used to make bullish predictions about artificial general intelligence , they’ve become quieter lately. Peter Thiel — the tech billionaire and rumored vampire — says Silicon Valley big brains have lost enthusiasm for AGI.
“Elon’s not talking about it anymore and Larry [Page] is off to Fiji and doesn’t seem to be working on it quite as hard,” Thiel said at a recent event .
Thiel described Musk as “a weathervane for the zeitgeist,” who’s stopped talking about AGI because interest has declined.
Scientists are also increasingly skeptical. A recent study paper posited that AGI is “in principle impossible,” while other researchers have mocked the term’s proponents.
“I have yet to come across work on AGI that I can take seriously,” tweeted Abeba Birhane, a cognitive scientist based at University College Dublin.
The path towards AGI increasingly appears — at best — a long one.
Imbuing machines with human-like intelligence remains immensely challenging. As Melanie Mitchell, a computer science professor at Portland State University noted in a preprint paper published last year on arXiv :
Critics fear that another AI winter is coming . Hyping AGI helped spurred enormous investment in artificial intelligence, but overconfident predictions could be detrimental to progress in the field — if the real-world advances prove disappointing.
Want to know when the pandemic will be over? Computer science may have the answer
In early 2022, nearly two years after Covid was declared a pandemic by the World Health Organization, experts are mulling a big question : when is a pandemic “over”?
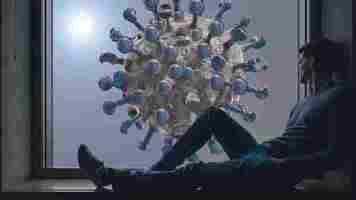
So, what’s the answer? What criteria should be used to determine the “end” of Covid’s pandemic phase? These are deceptively simple questions and there are no easy answers.
I am a computer scientist who investigates the development of ontologies. In computing, ontologies are a means to formally structure knowledge of a subject domain, with its entities, relations, and constraints, so that a computer can process it in various applications and help humans to be more precise.
Ontologies can discover knowledge that’s been overlooked until now: in one instance , an ontology identified two additional functional domains in phosphatases (a group of enzymes) and a novel domain architecture of a part of the enzyme. Ontologies also underlie Google’s Knowledge Graph that’s behind those knowledge panels on the right-hand side of a search result.
Applying ontologies to the questions I posed at the start is useful. This approach helps to clarify why it is difficult to specify a cut-off point at which a pandemic can be declared “over”. The process involves collecting definitions and characterizations from domain experts, like epidemiologists and infectious disease scientists, consulting relevant research and other ontologies, and investigating the nature of what entity “X” is.
“X”, here, would be the pandemic itself – not a mere shorthand definition, but looking into the properties of that entity. Such a precise characterization of the “X” will also reveal when an entity is “not an X”. For instance, if X = house, a property of houses is that they all must have a roof; if some object doesn’t have a roof, it definitely isn’t a house.
With those characteristics in hand, a precise, formal specification can be formulated, aided by additional methods and tools. From that, the what or when of “X” – the pandemic is over or it is not – would logically follow. If it doesn’t, at least it will be possible to explain why things are not that straightforward.
This sort of precision complements health experts’ efforts, helping humans to be more precise and communicate more precisely. It forces us to make implicit assumptions explicit and clarifies where disagreements may be.
Definitions and diagrams
I conducted an ontological analysis of “pandemic”. First, I needed to find definitions of a pandemic.
Informally, an epidemic is an occurrence during which there are multiple instances of an infectious disease in organisms, for a limited duration of time, that affects a community of said organisms living in some region. A pandemic, as a minimum, extends the region where the infections take place.
Next, I drew from existing foundational ontologies. This contains generic categories like “object”, “process”, and “quality”. I also used domain ontologies, which contain entities specific to a subject domain, like infectious diseases. Among other resources, I consulted the Infectious Disease Ontology and the Descriptive Ontology for Linguistic and Cognitive Engineering .
First, I aligned “pandemic” to a foundational ontology, using a decision diagram to simplify the process. This helped to work out what kind of thing and generic category “pandemic” is:
(1) Is [pandemic] something that is happening or occurring? Yes (perdurant, i something that unfolds in time, rather than be wholly present).
(2) Are you able to be present or participate in [a pandemic]? Yes (event).
(3) Is [a pandemic] atomic, i has no subdivisions and has a definite endpoint? No (accomplishment).
The word “accomplishment” may seem strange here. But, in this context, it makes clear that a pandemic is a temporal entity with a limited lifespan and will evolve – that is, cease to be a pandemic and evolve back to epidemic , as indicated in this diagram.
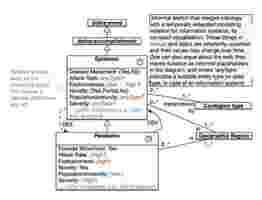
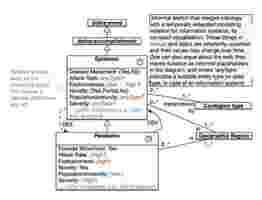
Characteristics
Next, I examined a pandemic’s characteristics described in the literature. A comprehensive list is described in a paper by US infectious disease specialists published in 2009 during the global H1N1 influenza virus outbreak. They collated eight characteristics of a pandemic.
I listed them and assessed them from an ontological perspective:
Properties with imprecise boundaries annoy epidemiologists because they may lead to different outcomes of their prediction models . But from my ontologist’s viewpoint, we’re getting somewhere with these properties. From the computational side, automated reasoning with fuzzy features is possible.
COVID, at least early in 2020, easily ticked all eight boxes. A suitably automated reasoner would have classified that situation as a pandemic. But now, in early 2022? Severity (point 8) has largely decreased and immunity (point 4) has risen. Point 5 – are there worse variants of concern to come – is the million-dollar question. More ontological analysis is needed.
Highlighting the difficulties
Ontologically speaking, then, a pandemic is an event (“accomplishment”) that unfolds in time. To be classified as a pandemic, there are a number of features that aren’t all crisp and for which the imprecise boundaries haven’t all been set. Conversely, it implies that classifying the event as “not a pandemic” is just as imprecise.
This isn’t a full answer as to what a pandemic is ontologically, but it does shed light on the difficulties of calling it “over” – and illustrates well that there will be disagreement about it.
This article by Maria Keet , Associate professor in Computer Science, University of Cape Town is republished from The Conversation under a Creative Commons license. Read the original article .
What to check about a Python machine learning book before buying it
With so many books on Python machine learning, making a choice is becoming increasingly difficult. You’re investing both your time and money to learn something that can open new career paths for you. It would a disappointment to get halfway through a 700-page machine learning book to realize it’s not for you.
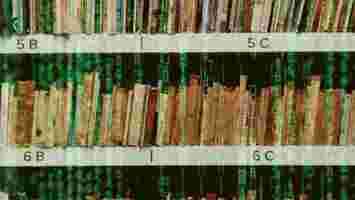
Having read and reviewed many books on Python machine learning , I can attest that every volume is unique in its own right. And with so many books having similar titles, the choice can be confusing, especially if you’re at the beginning of your machine learning journey.
Here are three things that will help you better evaluate a Python machine learning book before buying it.
The prerequisites
Every book on programming has a section in the preface or intro where the authors describe the book’s intended audience. This part is usually titled “prerequisites” or “who should read this book.” If you’re at a bookstore, pick up the book and flip to this section. If you’re buying online, Amazon and other online stores let you view the first few pages of the book, including the prerequisites section.
Here are a few things that are worth looking out for in the prerequisites section:
Python skills: Some books use simple code snippets just to prove a concept, while others make use of advanced Python features such as list comprehensions, slicing, with statements , parameter unpacking, and more. While it’s not fair to expect the book to create a comprehensive list of all techniques it will use, it should at least mention the level of skills you need. (Most machine learning books presume you have a basic understanding of data science and Python.)
Python libraries: Python machine learning books usually use ScikitLearn (and sometimes SciPy) to implement algorithms. Books on deep learning cover TensorFlow, Keras, and PyTorch. But these books also make use of scientific libraries such as Numpy, Pandas, and Matplotlib to load and manipulate data. Some books will cover these libraries while others will tell you that you should already have experience with them. (To be clear, these libraries have dedicated books that span several hundred pages.)
Python tools: Most Python machine learning developers prefer the Jupyter Notebook, a web-based interface that lets you code and test your algorithms in one place and save the results in HTML format. If a book will be using Jupyter, it should state whether it will take you through the installation and setup.
Math skills: Under the hood, machine learning involves a great deal of linear algebra, calculus, and statistics . Some books will try to describe the mechanics of machine learning algorithms through conceptual descriptions and drawings. Others will just spill the mathematical equations and let you figure it out for yourself (and some books will try to bridge the gap between the two with step-by-step description of the algorithms). Most books tell you how much math skills you need to read the book.
If a book doesn’t clearly state the requirements, don’t worry. We have other ways to find out.
The table of contents
A look at the table of contents will give a good picture of how deep it goes in different areas of machine learning and Python libraries. Most online bookstores let you look at the table of contents before buying the book. The table of contents will help you fill some of the gaps if the prerequisites section doesn’t explicitly state what you need to know before reading the book.
Here are a few things to look for in the table of contents:
Basics: Every book on machine learning starts with the basics. Advanced books tend to skim over this part and summarize it in a single chapter. Beginner books usually spend several chapters discussing the basics of machine learning, mathematics, data preprocessing and data types, and the machine learning pipeline.
Algorithms: Beginner books usually discuss algorithms in terms of the categories they fall into (regression, classification, clustering) and high-level concepts (supervised vs unsupervised learning, ensemble learning, hyperparameter tuning, dimensionality reduction). Advanced books usually have more granular chapters on specific types of algorithms (support vector machines, decision trees, principal component analysis, different clustering methods, etc.)
Python libraries: Advanced and intermediate books don’t discuss Python libraries such as NumPy and Matplotlib. Beginner books have sections that explain how to use these libraries to load, manipulate, and visualize data.
Projects: If this is your first book on Python machine learning, look for chapters that show end-to-end machine learning projects or examples on integrating machine learning into other applications. These hands-on, practical chapters will give you an idea of how machine learning fits into the general software development lifecycle. For instance, Hands-On Machine Learning with Scikit-Learn, Keras, and TensorFlow: Concepts, Tools, and Techniques to Build Intelligent Systems, 2 nd Edition has a chapter that takes you through the entire machine learning pipeline with a very practical example before going into the nitty-gritty of algorithm types.
Extras: Take a look at the final chapters and appendices. This is where you’ll find some of the unique gems the book has to offer, such as math and Python refreshers, using cloud hosting services, and introduction to big data platforms such as Hadoop and Apache Spark. Using cloud platforms and big data stores have complete educational volumes, but the knowledge provided in these books can give you directions on where to look next in your exploration of machine learning.
The code repo
While you can’t judge a machine learning book by its cover, in my experience, its code samples tell a lot about the book’s quality and its intended audience. Most Python machine learning books come with an accompanying GitHub repository, where you can get access to the code samples. The address to the repository is usually written in the book’s introduction, but you can also find it by googling the book’s title along with GitHub.
The official GitHub of the book is usually filed under the profile of the book’s author or the publisher. Make sure you don’t land on fake repos.
GitHub repositories of Python machine learning books have different structures. In general, you should see one folder for the notebooks and code samples of each chapter, and separate folders for assets such as datasets and images.
Open one of the chapter folders and examine its contents. Here are a few things that can determine the quality of the code:
Format: Does the book provide its samples in Jupyter Notebook format or plain Python code files? Jupyter Notebooks give a much richer experience. Even if you don’t run the code, they show you the results of the experiments (values, plot charts, etc.) if the authors have run them before uploading them.
Detail: Jupyter also allows for adding non-code information. Good books usually use Jupyter’s features to add details such as setup instructions, subtitles, and other information that help you correlate each code block to its corresponding place in the book.
Comments: I don’t expect a book’s code samples to be commented in detail, but there should at least be some minimal comments that show the basic steps of the experiment. Most code files also include lines that have been omitted in the book for the sake of brevity. Good books usually mark these sections in the comments.
Aside from the quality, the code files provide some good hints on the audience of the book in case the prerequisite section doesn’t provide enough information:
Examining the contents of one or two code sample files should determine the kind of Python skills you need. If you see things such as class inheritance, operator overloading, parameter unpacking, and nested list comprehensions, then the authors probably assume you’re a seasoned Python coder. Other books avoid advanced constructions for the sake of making it accessible to a wider audience.
Look at a few of the examples the book explores. Some books use housing prices, customer sales, and other real-world data to describe machine learning algorithms. These examples are usually more accessible to beginner audiences because they directly correlate with a real-world situation. They usually build on these examples through one or more Jupyter Notebooks and chapters to help you understand the machine learning pipeline. Intermediate level books use benchmark data sets such as CIFAR, MNIST, Iris, or wine data. These are lightweight datasets that can help understand the importance of different configurations and hyperparameters, but are not very good for end-to-end examples. Other books might use dataset generator functions in Python libraries such as make_blobs, make_circles, and make_classification , which have no conceptual value but are good for showing how algorithms work.
Final thoughts
No single book to make you a master of Python machine learning. But hopefully, these tips will help you make the best of your time and money and have a smooth path in teaching yourself machine learning.
All this said, here are a few recommendations based on what we’ve reviewed before:
If you’re new to the world of data science and machine learning but know the basics of Python, try one of these books .
If you’re comfortable with data science concepts and Python libraries (Numpy, Matplotlib), try Hands-on Machine Learning (introduced above) or Python Machine Learning, Third Edition . If you’re more comfortable with video tutorials, Udemy’s Machine Learning A-Z online course is a great experience.
If you’re already comfortable with the machine learning math and Python libraries, read Machine Learning Algorithms, Second Edition to deepen your knowledge and skills.
This article was originally published by Ben Dickson on TechTalks , a publication that examines trends in technology, how they affect the way we live and do business, and the problems they solve. But we also discuss the evil side of technology, the darker implications of new tech and what we need to look out for. You can read the original article here .