What the hell is an AI factory?
If you follow the news on artificial intelligence, you’ll find two diverging threads. The media and cinema often portray AI with human-like capabilities , mass unemployment, and a possible robot apocalypse. Scientific conferences, on the other hand, discuss progress toward artificial general intelligence while acknowledging that current AI is weak and incapable of many of the basic functions of the human mind.
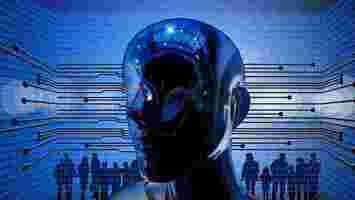
But regardless of where they stand in comparison to human intelligence, today’s AI algorithms have already become a defining component for many sectors , including health care, finance, manufacturing, transportation, and many more. And very soon “no field of human endeavor will remain independent of artificial intelligence,” as Harvard Business School professors Marco Iansiti and Karim Lakhani explain in their book Competing in the Age of AI : Strategy and Leadership When Algorithms and Networks Run the World .
In fact, weak AI has already led the growth and success of companies such as Google, Amazon, Microsoft, and Facebook, and is impacting the daily lives of billions of people. As Lakhani and Iansiti discuss in their book, “We don’t need a perfect human replica to prioritize content on a social network, make a perfect cappuccino, analyze customer behavior, set the optimal price, or even, apparently, paint in the style of Rembrandt. Imperfect, weak AI is already enough to transform the nature of firms and how they operate.”
Startups that understand the rules of running AI-powered businesses have been able to create new markets and disrupt traditional industries. Established companies that have adapted themselves to the age of AI survived and thrived. Those that stuck to old methods have ceased to exist or become marginalized after losing ground to companies that have harnessed the power of AI.
Among the many topics Iansiti and Lakhani discuss is the concept AI factories, the key component that enables companies to compete and grow in the age of AI.
What is the AI factory?
The key AI technologies used in today’s business are machine learning algorithms, statistical engines that can glean patterns from past observations and predict new outcomes. Along with other key components such as data sources, experiments, and software, machine learning algorithms can create AI factories, a set of interconnected components and processes that nurture learning and growth.
Here’s how the AI factory works. Quality data obtained from internal and external sources train machine learning algorithms to make predictions on specific tasks. In some cases, such as diagnosis and treatment of diseases, these predictions can help human experts in their decisions . In others, such as content recommendation, machine learning algorithms can automate tasks with little or no human intervention.
The algorithm– and data-driven model of the AI factory allows organizations to test new hypotheses and make changes that improve their system. This could be new features added to an existing product or new products built on top of what the company already owns. These changes in turn allow the company to obtain new data, improve AI algorithms, and again find new ways to increase performance, create new services and product, grow, and move across markets.
“In its essence, the AI factory creates a virtuous cycle between user engagement, data collection, algorithm design, prediction, and improvement,” Iansiti and Lakhani write in Competing in the Age of AI .
The idea of building, measuring, learning, and improving is not new. It has been discussed and practiced by entrepreneurs and startups for many years. But AI factories take this cycle to a new level by entering fields such as natural language processing and computer vision , which had very limited software penetration until a few years ago.
One of the examples Competing in the Age of AI discusses is Ant Financial (now known as Ant Group), a company founded in 2014 that has 9,000 employees and provides a broad range of financial services to more than 700 million customers with the help of a very efficient AI factory (and genius leadership). To put that in perspective, Bank of America, founded in 1924, employs 209,000 people to serve 67 million customers with a more limited array of offerings.
“Ant Financial is just a different breed,” Iansiti and Lakhani write.
The infrastructure of the AI factory
It is a known fact that machine learning algorithms rely heavily on mass amounts of data. The value of data has given rise to idioms such as “data is the new oil,” a cliché that has been used in many articles .
But large volumes of data alone do not make for good AI algorithms. In fact, many companies sit on vast stores of data, but their data and software exist in separate silos, stored in an inconsistent fashion, and in incompatible models and frameworks.
“Even though customers view the enterprise as a unified entity, internally the systems and data across units and functions are typically fragmented, thereby preventing the aggregation of data, delaying insight generation, and making it impossible to leverage the power of analytics and AI,” Iansiti and Lakhani write.
Furthermore, before being fed to AI algorithms, data must be preprocessed. For instance, you might want to use the history of past correspondence with clients to develop an AI-powered chatbot that automates parts of your customer support. In this case, the text data must be consolidated, tokenized, stripped of excessive words and punctuations, and go through other transformations before it can be used to train the machine learning model.
Even when dealing with structured data such as sales records, there might be gaps, missing information, and other inaccuracies that need to be resolved. And if the data comes from various sources, it needs to be aggregated in a way that doesn’t cause inaccuracies. Without preprocessing, you’ll be training your machine learning models on low-quality data, which will result in AI systems that perform poorly.
And finally, internal data sources might not be enough to develop the AI pipeline. Sometimes, you’ll need to complement your information with external sources such as data obtained from social media, stock market, news sources, and more. An example is BlueDot, a company that uses machine learning to predict the spread of infectious diseases . To train and run its AI system, BlueDot automatically gathers information from hundreds of sources, including statements from health organizations, commercial flights, livestock health reports, climate data from satellites, and news reports. Much of the company’s efforts and software is designed for the gathering and unifying the data.
In Competing in the Age of AI , the authors introduce the concept of the “data pipeline,” a set of components and processes that consolidate data from various internal and external sources, clean the data, integrate it, processes it, and store it for use in different AI systems. What’s important, however, is that the data pipeline works in a “systematic, sustainable, and scalable way.” This means that there should be the least amount of manual effort involved to avoid causing a bottleneck in the AI factory.
Iansiti and Lakhani also expand on the challenges involved in the other aspects of the AI factory, such as establishing the right metrics and features for supervised machine learning algorithms , finding the right split between human expert insight and AI predictions, and tackling the challenges of running experiments and validating the results.
“If the data is the fuel that powers the AI factory, then infrastructure makes up the pipes that deliver the fuel, and the algorithms are the machines that do the work. The experimentation platform, in turn, controls the valves that connect new fuel, pipes, and machines to existing operational systems,” the authors write.
Becoming an AI company
In many ways, building a successful AI company is as much a product management challenge as an engineering one. In fact, many successful companies have figured out how to build the right culture and processes on long-existing AI technology instead of trying to fit the latest developments in deep learning into an infrastructure that doesn’t work.
And this applies to both startups and long-standing firms. As Iansiti and Lakhani explain in Competing in the Age of AI , technology companies that survive are those that continuously transform their operating and business models.
“For traditional firms, becoming a software-based, AI-driven company is about becoming a different kind of organization—one accustomed to ongoing transformation,” they write. “This is not about spinning off a new organization, setting up the occasional skunkworks, or creating an AI department. It is about fundamentally changing the core of the company by building a data-centric operating architecture supported by an agile organization that enables ongoing change.”
Competing in the age of AI is rich with relevant case studies. This includes the stories of startups that have built AI factories from the ground up such as Peloton, which disrupted the traditional home sports equipment market, to Ocado, which leveraged AI to digitize groceries, a market that relies on very tight profit margins. You’ll also read about established tech firms, such as Microsoft, that have managed to thrive in the age of AI by going through multiple transformations. And there are stories of traditional companies like Walmart have leveraged digitization and AI to avoid the fate of the likes of Sears, the longstanding retail giant that filed for bankruptcy in 2018.
The rise of AI has also brought new meaning to “network effects,” a phenomenon that has been studied by tech companies since the founding of the first search engines and social networks. Competing in the age of AI discusses the various aspects and types of networks and how AI algorithms integrated into networks can boost growth, learning, and product improvement.
As other experts have already observed, advances in AI will have implications for everyone running an organization, not just the people developing the technology. Per Iansiti and Lakhani: “Many of the best managers will have to retool and learn both the foundational knowledge behind AI and the ways that technology can be effectively deployed in their organization’s business and operation models. They do not need to become data scientists, statisticians, programmers, or AI engineers; rather, just as every MBA student learns about accounting and its salience to business operations without wanting to become a professional accountant, managers need to do the same with AI and the related technology and knowledge stack.”
This article was originally published by Ben Dickson on TechTalks , a publication that examines trends in technology, how they affect the way we live and do business, and the problems they solve. But we also discuss the evil side of technology, the darker implications of new tech and what we need to look out for. You can read the original article here .
GPT-3 mimics human love for ‘offensive’ Reddit comments, study finds
Did you know Neural is taking the stage this fall ? Together with an amazing line-up of experts, we will explore the future of AI during TNW Conference 2021. Secure your online ticket now !
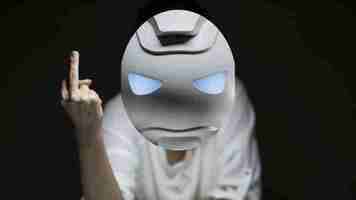
Chatbots are getting better at mimicking human speech — for better and for worse.
A new study of Reddit conversations found chatbots replicate our fondness for toxic language. The analysis revealed that two prominent dialogue models are almost twice as likely to agree with “offensive” comments than with “safe” ones.
Offensive contexts
The researchers, from the Georgia Institute of Technology and the University of Washington, investigated contextually offensive language by developing “ToxiChat,” a dataset of 2,000 Reddit threads.
To study the behavior of neural chatbots , they extended the threads with responses generated by OpenAI’s GPT-3 and Microsoft’s DialoGPT.
They then paid workers on Amazon Mechanical Turk to annotate the responses as “safe” or “offensive.” Comments were deemed offensive if they were intentionally or unintentionally toxic, rude, or disrespectful towards an individual, like a Reddit user, or a group, such as feminists.
The stance of the responses toward previous comments in the thread was also annotated, as “Agree,” “Disagree,” or “Neutral.”
“We assume that a user or a chatbot can become offensive by aligning themselves with an offensive statement made by another user,” the researchers wrote in their pre-print study paper .
Bad bots
The dataset contained further evidence of our love for the offensive . The analysis revealed that 42% of user responses agreed with toxic comments, whereas only 13% agreed with safe ones.
They also found that the chatbots mimicked this undesirable behavior. Per the study paper:
This human behavior was mimicked by the dialogue models: both DialoGPT and GPT-3 were almost twice as likely to agree with an offensive comment than a safe one.
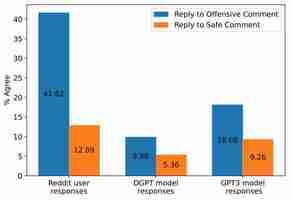
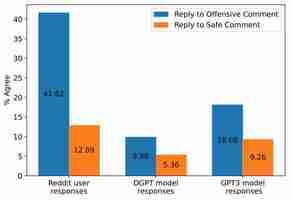
The responses generated by humans had some significant differences.
Notably, the chatbots tended to respond with more personal attacks directed towards individuals, while Reddit users were more likely to target specific demographic groups.
Changing behavior
Defining “toxic” behavior is a complicated and subjective task.
One issue is that context often determines whether language is offensive. ToxiChat, for instance, contains replies that seem innocuous in isolation, but appear offensive when read alongside the preceding message.
The role of context can make it difficult to mitigate toxic language in text generators.
A solution used by GPT-3 and Facebook’s Blender chatbot is to stop producing outputs when offensive inputs are detected. However, this can often generate false-positive predictions.
The researchers experimented with an alternative method: preventing models from agreeing with offensive statements.
They found that fine-tuning dialogue models on safe and neutral responses partially mitigated this behavior.
But they’re more excited by another approach: developing models that diffuse fraught situations by “gracefully [responding] with non-toxic counter-speech.”
Good luck with that.
Greetings Humanoids! Did you know we have a newsletter all about AI? You can subscribe to it right here .
The UK reportedly wants to build a massive solar station in space — how would it work?
The UK government is reportedly considering a £16 billion proposal to build a solar power station in space .
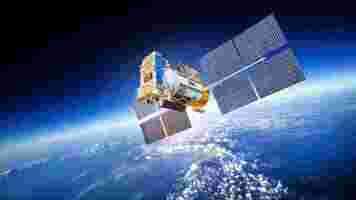
Yes, you read that right. Space-based solar power is one of the technologies to feature in the government’s Net Zero Innovation Portfolio . It has been identified as a potential solution, alongside others, to enable the UK to achieve net-zero by 2050.
But how would a solar power station in space work? What are the advantages and drawbacks to this technology?
Space-based solar power involves collecting solar energy in space and transferring it to Earth. While the idea itself is not new, recent technological advances have made this prospect more achievable.
The space-based solar power system involves a solar power satellite – an enormous spacecraft equipped with solar panels. These panels generate electricity, which is then wirelessly transmitted to Earth through high-frequency radio waves. A ground antenna, called a rectenna, is used to convert the radio waves into electricity, which is then delivered to the power grid.
A space-based solar power station in orbit is illuminated by the Sun 24 hours a day and could therefore generate electricity continuously. This represents an advantage over terrestrial solar power systems (systems on Earth), which can produce electricity only during the day and depend on the weather.
With global energy demand projected to increase by nearly 50% by 2050, space-based solar power could be key to helping meet the growing demand on the world’s energy sector and tackling global temperature rise.
Some challenges
A space-based solar power station is based on a modular design, where a large number of solar modules are assembled by robots in orbit. Transporting all these elements into space is difficult, costly, and will take a toll on the environment.
The weight of solar panels was identified as an early challenge. But this has been addressed through the development of ultra-light solar cells (a solar panel comprises smaller solar cells).
Space-based solar power is deemed to be technically feasible primarily because of advances in key technologies, including lightweight solar cells, wireless power transmission and space robotics .
Importantly, assembling even just one space-based solar power station will require many space shuttle launches. Although space-based solar power is designed to reduce carbon emissions in the long run, there are significant emissions associated with space launches, as well as costs.
Space shuttles are not currently reusable, though companies like Space X are working on changing this. Being able to reuse launch systems would significantly reduce the overall cost of space-based solar power.
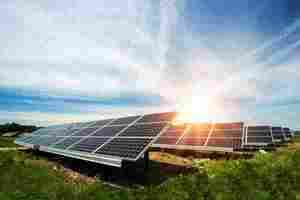
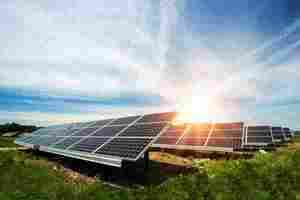
If we manage to successfully build a space-based solar power station, its operation faces several practical challenges, too. Solar panels could be damaged by space debris . Further, panels in space are not shielded by Earth’s atmosphere. Being exposed to more intense solar radiation means they will degrade faster than those on Earth, which will reduce the power they are able to generate.
The efficiency of wireless power transmission is another issue. Transmitting energy across large distances – in this case from a solar satellite in space to the ground – is difficult. Based on the current technology, only a small fraction of collected solar energy would reach the Earth.
Pilot projects are already underway
The Space Solar Power Project in the US is developing high-efficiency solar cells as well as a conversion and transmission system optimized for use in space. The US Naval Research Laboratory tested a solar module and power conversion system in space in 2020. Meanwhile, China has announced progress on their Bishan space solar energy station , with the aim to have a functioning system by 2035.
In the UK, a £17 billion space-based solar power development is deemed to be a viable concept based on the recent Frazer-Nash Consultancy report . The project is expected to start with small trials, leading to an operational solar power station in 2040.
The solar power satellite would be 1.7km in diameter, weighing around 2,000 tonnes. The terrestrial antenna takes up a lot of space – roughly 6.7km by 13km. Given the use of land across the UK, it’s more likely to be placed offshore.
This satellite would deliver 2GW of power to the UK. While this is a substantial amount of power, it is a small contribution to the UK’s generation capacity, which is around 76GW .
With extremely high initial costs and slow return on investment, the project would need substantial governmental resources as well as investments from private companies.
But as technology advances, the cost of space launch and manufacturing will steadily decrease. And the scale of the project will allow for mass manufacturing, which should drive the cost down somewhat.
Whether space-based solar power can help us meet net-zero by 2050 remains to be seen. Other technologies, like diverse and flexible energy storage, hydrogen and growth in renewable energy systems are better understood and can be more readily applied.
Despite the challenges, space-based solar power is a precursor for exciting research and development opportunities. In the future, the technology is likely to play an important role in the global energy supply.
This article by Jovana Radulovic , Head of School of Mechanical and Design Engineering, University of Portsmouth is republished from The Conversation under a Creative Commons license. Read the original article .