What will happen when we reach the AI singularity?
Should you feel bad about pulling the plug on a robot or switch off an artificial intelligence algorithm? Not for the moment. But how about when our computers become as smart—or smarter—than us?
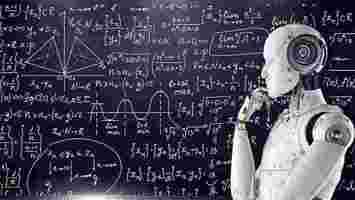
Debates about the consequences of artificial general intelligence (AGI) are almost as old as the history of AI itself. Most discussions depict the future of artificial intelligence as either Terminator -like apocalypse or Wall-E -like utopia. But what’s less discussed is how we will perceive, interact with, and accept artificial intelligence agents when they develop traits of life, intelligence, and consciousness.
In a recently published essay, Borna Jalsenjak, scientist at Zagreb School of Economics and Management, discusses super-intelligent AI and analogies between biological and artificial life. Titled “The Artificial Intelligence Singularity: What It Is and What It Is Not,” his work appears in Guide to Deep Learning Basics , a collection of papers and treatises that explore various historic, scientific, and philosophical aspects of artificial intelligence.
Jalsenjak takes us through the philosophical anthropological view of life and how it applies to AI systems that can evolve through their own manipulations. He argues that “thinking machines” will emerge when AI develops its own version of “life,” and leaves us with some food for thought about the more obscure and vague aspects of the future of artificial intelligence.
AI singularity
Singularity is a term that comes up often in discussions about general AI. And as is wont with everything that has to do with AGI, there’s a lot of confusion and disagreement on what the singularity is. But a key thing that most scientists and philosophers agree that it is a turning point where our AI systems become smarter than ourselves. Another important aspect of the singularity is time and speed: AI systems will reach a point where they can self-improve in a recurring and accelerating fashion.
“Said in a more succinct way, once there is an AI which is at the level of human beings and that AI can create a slightly more intelligent AI, and then that one can create an even more intelligent AI, and then the next one creates even more intelligent one and it continues like that until there is an AI which is remarkably more advanced than what humans can achieve,” Jalsenjak writes.
To be clear, the artificial intelligence technology we have today, known as narrow AI , is nowhere near achieving such feat. Jalšenjak describes current AI systems as “domain-specific” such as “AI which is great at making hamburgers but is not good at anything else.” On the other hand, the kind of algorithms that is the discussion of AI singularity is “AI that is not subject-specific, or for the lack of a better word, it is domainless and as such it is capable of acting in any domain,” Jalsenjak writes.
This is not a discussion about how and when we’ll reach AGI. That’s a different topic, and also a focus of much debate, with most scientists in the belief that human-level artificial intelligence is at least decades away . Jalsenjack rather speculates of how the identity of AI (and humans) will be defined when we actually get there, whether it be tomorrow or in a century.
Is artificial intelligence alive?
There’s great tendency in the AI community to view machines as humans , especially as they develop capabilities that show signs of intelligence. While that is clearly an overestimation of today’s technology, Jasenjak also reminds us that artificial general intelligence does not necessarily have to be a replication of the human mind.
“That there is no reason to think that advanced AI will have the same structure as human intelligence if it even ever happens, but since it is in human nature to present states of the world in a way that is closest to us, a certain degree of anthropomorphizing is hard to avoid,” he writes in his essay’s footnote.
One of the greatest differences between humans and current artificial intelligence technology is that while humans are “alive” (and we’ll get to what that means in a moment), AI algorithms are not.
“The state of technology today leaves no doubt that technology is not alive,” Jalsenjak writes, to which he adds, “What we can be curious about is if there ever appears a superintelligence such like it is being predicted in discussions on singularity it might be worthwhile to try and see if we can also consider it to be alive.”
Albeit not organic, such artificial life would have tremendous repercussions on how we perceive AI and act toward it.
What would it take for AI to come alive?
Drawing from concepts of philosophical anthropology, Jalsenjak notes that living beings can act autonomously and take care of themselves and their species, what is known as “immanent activity.”
“Now at least, no matter how advanced machines are, they in that regard always serve in their purpose only as extensions of humans,” Jalsenjak observes.
There are different levels to life, and as the trend shows, AI is slowly making its way toward becoming alive. According to philosophical anthropology, the first signs of life take shape when organisms develop toward a purpose, which is present in today’s goal-oriented AI. The fact that the AI is not “aware” of its goal and mindlessly crunches numbers toward reaching it seems to be irrelevant, Jalsenjak says, because we consider plants and trees as being alive even though they too do not have that sense of awareness.
Another key factor for being considered alive is a being’s ability to repair and improve itself, to the degree that its organism allows. It should also produce and take care of its offspring. This is something we see in trees, insects, birds, mammals, fish, and practically anything we consider alive. The laws of natural selection and evolution have forced every organism to develop mechanisms that allow it to learn and develop skills to adapt to its environment, survive, and ensure the survival of its species.
On child-rearing, Jalsenjak posits that AI reproduction does not necessarily run in parallel to that of other living beings. “Machines do not need offspring to ensure the survival of the species. AI could solve material deterioration problems with merely having enough replacement parts on hand to swap the malfunctioned (dead) parts with the new ones,” he writes. “Live beings reproduce in many ways, so the actual method is not essential.”
When it comes to self-improvement, things get a bit more subtle. Jalsenjak points out that there is already software that is capable of self-modification, even though the degree of self-modification varies between different software.
Today’s machine learning algorithms are, to a degree, capable of adapting their behavior to their environment. They tune their many parameters to the data collected from the real-world, and as the world changes, they can be retrained on new information. For instance, the coronavirus pandemic disrupted may AI systems that had been trained on our normal behavior. Among them are facial recognition algorithms that can no longer detect faces because people are wearing masks. These algorithms can now retune their parameters by training on images of mask-wearing faces. Clearly, this level of adaptation is very small when compared to the broad capabilities of humans and higher-level animals, but it would be comparable to, say, trees that adapt by growing deeper roots when they can’t find water at the surface of the ground.
An ideal self-improving AI, however, would be one that could create totally new algorithms that would bring fundamental improvements. This is called “recursive self-improvement” and would lead to an endless and accelerating cycle of ever-smarter AI. It could be the digital equivalent of the genetic mutations organisms go through over the span of many many generations, though the AI would be able to perform it at a much faster pace.
Today, we have some mechanisms such as genetic algorithms and grid-search that can improve the non-trainable components of machine learning algorithms (also known as hyperparameters). But the scope of change they can bring is very limited and still requires a degree of manual work from a human developer. For instance, you can’t expect a recursive neural network to turn into a Transformer through many mutations.
Recursive self-improvement, however, will give AI the “possibility to replace the algorithm that is being used altogether,” Jalsenjak notes. “This last point is what is needed for the singularity to occur.”
By analogy, looking at determined characteristics, superintelligent AIs can be considered alive, Jalsenjak concludes, invalidating the claim that AI is an extension of human beings. “They will have their own goals, and probably their rights as well,” he says, “Humans will, for the first time, share Earth with an entity which is at least as smart as they are and probably a lot smarter.”
Would you still be able to unplug the robot without feeling guilt?
Being alive is not enough
At the end of his essay, Jalsenjak acknowledges that the reflection on artificial life leaves many more questions. “Are characteristics described here regarding live beings enough for something to be considered alive or are they just necessary but not sufficient?” He asks.
Having just read I Am a Strange Loop by philosopher and scientist Douglas Hofstadter, I can definitely say no. Identity, self-awareness, and consciousness are other concepts that discriminate living beings from one another. For instance, is a mindless paperclip-builder robot that is constantly improving its algorithms to turn the entire universe into paperclips alive and deserving of its own rights?
Free will is also an open question. “Humans are co-creators of themselves in a sense that they do not entirely give themselves existence but do make their existence purposeful and do fulfill that purpose,” Jalsenjak writes. “It is not clear will future AIs have the possibility of a free will.”
And finally, there is the problem of the ethics of superintelligent AI. This is a broad topic that includes the kinds of moral principles AI should have, the moral principles humans should have toward AI, and how AIs should view their relations with humans.
The AI community often dismisses such topics, pointing out to the clear limits of current deep learning systems and the far-fetched notion of achieving general AI.
But like many other scientists , Jalsenjak reminds us that the time to discuss these topics is today, not when it’s too late. “These topics cannot be ignored because all that we know at the moment about the future seems to point out that human society faces unprecedented change,” he writes.
In the full essay, available at Springer , Jalsenjak provides in-depth details of artificial intelligence singularity and the laws of life. The complete book, Guide to Deep Learning Basics , provides more in-depth material about the philosophy of artificial intelligence.
This article was originally published by Ben Dickson on TechTalks , a publication that examines trends in technology, how they affect the way we live and do business, and the problems they solve. But we also discuss the evil side of technology, the darker implications of new tech and what we need to look out for. You can read the original article here .
AI devs claim they’ve created a robot that demonstrates a ‘primitive form of empathy’
Columbia University researchers have developed a robot that displays a “glimmer of empathy” by visually predicting how another machine will behave.
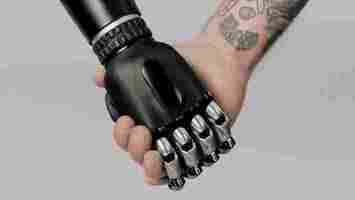
The robot learns to forecast its partner’s future actions and goals by observing a few video frames of its actions
The researchers first programmed the partner robot to move towards green circles in a playpen of around 3×2 feet in size. It would sometimes move directly towards a green circle spotted by its cameras, but if the circles were hidden by an obstacle, it would either roll towards a different circle or not move at all.
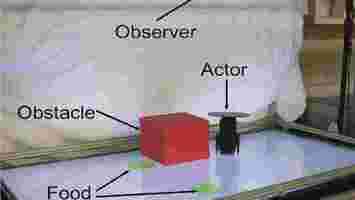
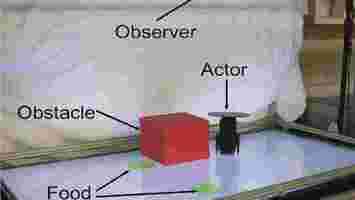
After the observer robot watched the actor’s behavior for roughly two hours, it started guessing its partner’s future movements. It eventually managed to predict the subject’s goal and path 98 out of 100 times.
Boyuan Chen, the lead author of the study, said the initial results were “very exciting:”
The team believes their approach could help pave a towards a robotic “Theory of Mind,” which humans use to understand other people’s thoughts and feelings.
“We hypothesize that such visual behavior modeling is an essential cognitive ability that will allow machines to understand and coordinate with surrounding agents, while sidestepping the notorious symbol grounding problem ,” the researchers said in their study paper .
The researchers admit that there are many limitations to their project. They note that the observer hasn’t handled complex actor logic and that they gave it a full overhead view, when in practice it would typically only have a first-person perspective or partial information.
They also warn that giving robots the ability to anticipate how humans think could lead them to manipulate our thoughts.
“We recognize that robots aren’t going to remain passive instruction-following machines for long,” said study lead Professor Hod Lipson.
“Like other forms of advanced AI, we hope that policymakers can help keep this kind of technology in check, so that we can all benefit.”
Nonetheless, the team believes the “visual foresight” they’ve demonstrated could deepen our understanding of human social behavior — and lay the foundations for more socially adept machines.
You can read their study paper in Nature Scientific Reports and find all their code and data at GitHub .
New AI tool that detects star flares could help us find habitable planets
A new AI system that detects flares erupting from stars could help astronomers find habitable planets, according to the tool’s inventors.
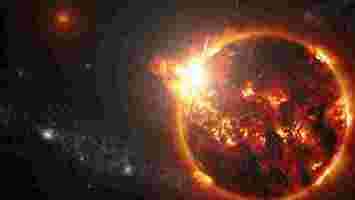
The neural network detects the light patterns of a stellar flare — which can incinerate the atmospheres of planets forming nearby. The frequency and location of the flares can therefore indicate the best places to search for habitable planets.
Astronomers normally look for the flares through a time-consuming process of analyzing measurements of star brightness by eye. The AI tool could make their work faster and more effective.
The researchers trained the neural network on a dataset of identified flares and not-flares, and then applied it to a dataset of more than 3,200 stars. It discovered more than 23,000 flares across thousands of young stars.
The researchers found that stars similar to our sun only have a few flares, which seem to decrease in number after around 50 million years.
“This is good for fostering planetary atmospheres – a calmer stellar environment means the atmospheres have a better chance of surviving,” said Adina Feinstein, a University of Chicago graduate student and first author on the paper.
However, cooler stars called red dwarfs tended to have more frequent flares, which could make it hard for the planets they host to retain any atmosphere.
The researchers now plan to modify the neural network so it can look for planets close to young stars.
“This will hopefully lead to a ‘rise of the machines’ where we can apply machine learning algorithms to find a bunch of exciting new planets using the same methods,” said UNSW Sydney’s Dr Ben Montet, co-author of the study.
You can read more on the study findings i n the Astronomical Journal and the Journal of Open Source Software .